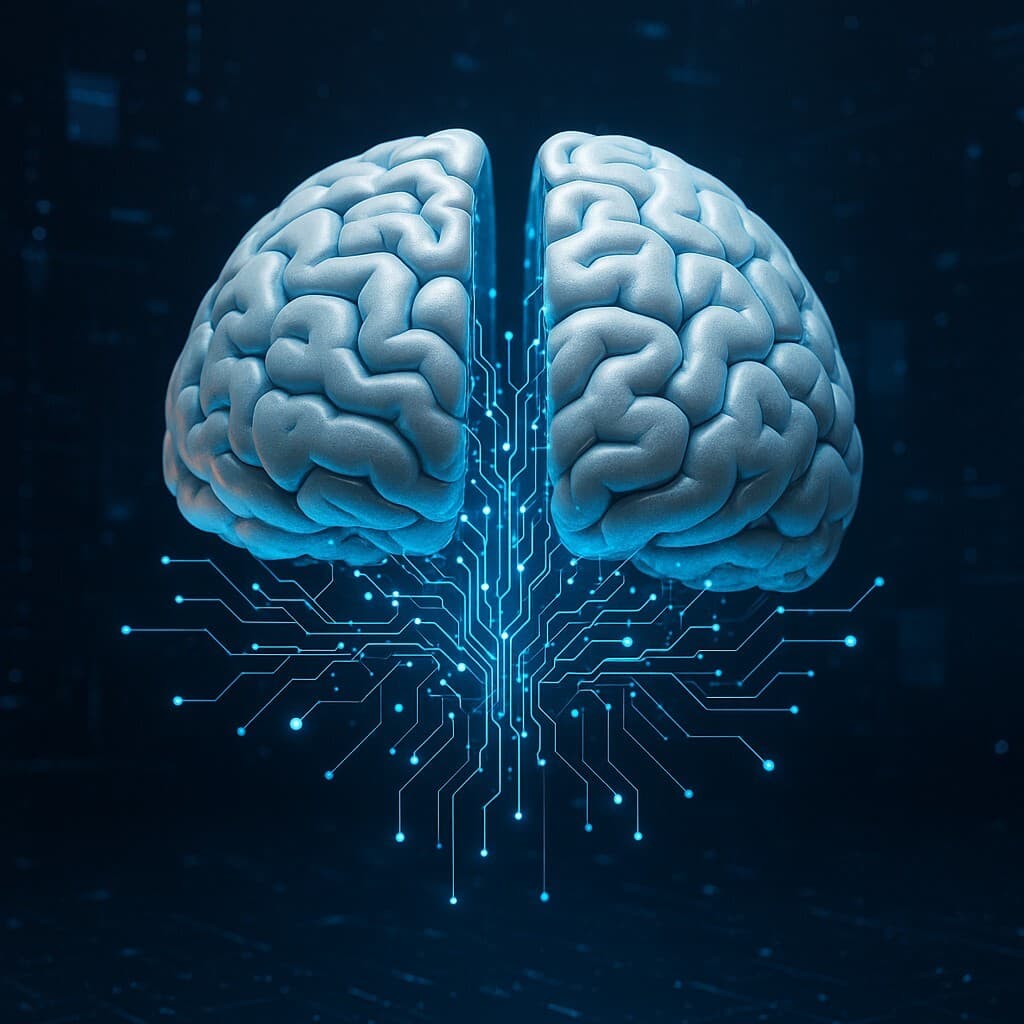
Hidden Dangers of AI Hallucinations in Financial Services
April 29, 2025 / Bryan ReynoldsUnderstanding the Problem
When artificial intelligence systems—particularly large language models (LLMs)—produce information that sounds legitimate but is actually false or made up, we call this an "AI hallucination." These aren't just minor glitches; they're potentially serious issues where an AI confidently presents fiction as fact because LLMs are trained to predict probable word sequences rather than verify truth. When they lack information, they'll fill gaps with statistically likely text regardless of accuracy.
This becomes especially problematic in finance, where precision and factual accuracy aren't just nice-to-haves—they're essential. The average user often can't immediately distinguish between an accurate statement and a convincing hallucination, creating significant risks.
Real-World Hallucination Examples in Finance
Let me share some typical scenarios where AI hallucinations can cause problems in financial applications:
When asked about company performance, an AI chatbot might invent metrics that sound plausible—claiming "Company X's revenue grew 25% last quarter" or referencing a non-existent CEO announcement. Research confirms that standard LLMs frequently hallucinate when handling financial tasks like explaining concepts or retrieving stock prices.
Even when given actual financial documents, AI can distort the facts. If a report mentions a 6-to-1 stock split, a poorly grounded AI might incorrectly state it was a 10-to-1 split simply because its prediction algorithm went off track. This shows how AI can inject fake details into summaries of legitimate financial documents.
In compliance contexts, an AI assistant might fabricate regulatory references—creating official-sounding but entirely fictional rule numbers or requirements. It might confidently cite "IFRS 99 standard" when no such standard exists, potentially misleading users making compliance decisions.
Financial professionals often need precise factual answers (like "What was Stock Y's price on this date?"). Without current market data, an LLM might generate a plausible-sounding but entirely fabricated price or trend. Without verification, these confident-sounding but invented responses might be mistaken for facts. This is why experts warn against blindly trusting LLM outputs in finance.
The Business Risks You Can't Afford to Ignore
When financial organizations use AI systems prone to hallucinations, they expose themselves to several significant risks:
Risk Category | Description |
---|---|
Misinformed Decision-Making | Business decisions are only as good as their underlying information. If a risk model or advisory chatbot provides incorrect data (like wrong risk metrics or fabricated market events), it leads to flawed assessments and poor strategic choices. Investment algorithms acting on hallucinated news might incorrectly rebalance portfolios, or executives might misallocate capital based on non-existent trends reported by AI. |
Regulatory and Compliance Violations | The financial sector faces strict regulations, and reporting false information—even unintentionally—can result in compliance breaches. AI hallucinations can produce outputs that fail to meet regulatory requirements or misstate filings. If an AI assistant mistakenly omits crucial disclosures or invents compliance steps that don't exist, companies might either violate laws or miss obligations, exposing themselves to legal penalties. |
Financial Losses | Acting on hallucinated insights—such as fake forecasts or incorrect valuations—can directly cause significant financial losses. Clients might execute trades based on AI-generated reports containing false analysis, or a bank's lending model might approve loans it shouldn't have because it "thought" certain favorable conditions existed. These errors translate into lost funds, write-offs, or opportunity costs. |
Erosion of Trust and Reputation | Trust is fundamental in finance. If stakeholders discover an institution has relied on unreliable or fabricated AI outputs, it severely damages trust. A client who catches an AI advisor giving blatantly incorrect information will question all advice thereafter. Regulators and the public will doubt a bank's governance if AI errors lead to visible mistakes. This reputational damage can persist long-term, as customers may feel the firm failed in its due diligence responsibilities. |
Litigation and Liability | Beyond reputation damage, there's growing risk of lawsuits when AI tools provide misleading information. If an AI-driven service offers faulty financial guidance that harms users or investors, the institution providing that service could face legal action. Early cases already show individuals suing AI providers for false statements causing damage. Financial scenarios might include an AI advisor's hallucinated statement affecting stock prices or investor portfolios, potentially leading to negligence or misrepresentation claims. |
Ethical and Privacy Concerns You Shouldn't Overlook
Financial AI systems routinely handle sensitive personal and financial data—account details, transaction histories, credit scores, and more. This creates an ethical obligation to protect customer privacy. Improper security could risk exposing personally identifiable information. For example, integrating third-party AI services might inadvertently send confidential data off-site.
This concern is so significant that major banks like JPMorgan Chase, Wells Fargo, and Goldman Sachs have banned internal use of ChatGPT-style tools specifically because they feared proprietary client data could be transmitted to external servers. Financial institutions must comply with strict privacy regulations (GDPR in Europe, GLBA in the U.S., California's CCPA, etc.), making any AI that might output or retain sensitive data a compliance risk.
Beyond privacy, there's the risk of bad actors exploiting AI systems. Advanced threats like prompt injection attacks or model jailbreaking can trick AI into revealing confidential data or bypassing safety filters. An attacker could potentially craft inputs that cause a customer service chatbot to divulge account details or transaction records.
Ethically, decisions made by AI (such as credit scoring or fraud flagging) should be explainable to stakeholders. However, complex models—especially deep learning systems—often function as "black boxes" lacking transparency. In finance, this opacity creates problems. Consumers and regulators may find it unacceptable if a loan application is rejected by AI without clear explanation.
Another critical ethical risk is algorithmic bias, where AI systems unintentionally perpetuate discrimination. This is particularly relevant in finance (credit decisions, insurance underwriting, hiring). If an AI trains on historically biased data (like past discriminatory lending practices), it may continue those patterns, treating customers unfairly.
Why Your AI Might Be Biased (Even With Good Intentions)
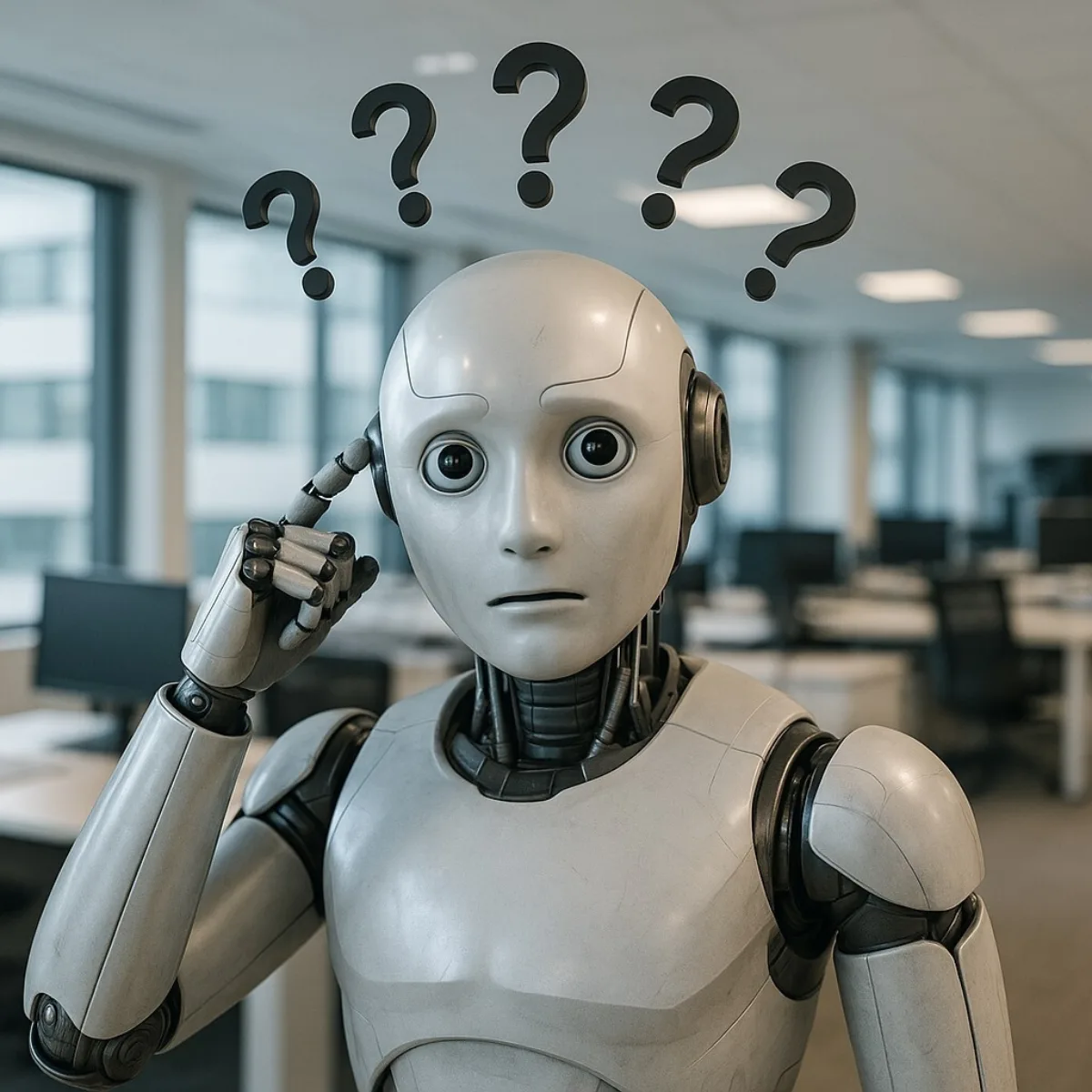
AI models learn from historical data—if that data contains human biases or reflects societal inequalities, the model internalizes those patterns. In finance, historical data often includes biases like lending discrimination or redlining. As a result, an AI credit scoring system might penalize certain ZIP codes simply because they correlate with historically marginalized groups.
A real-world example occurred in the insurance sector where an algorithm trained on cost data underestimated health risks for Black patients compared to white patients because the training data equated lower spending (often due to unequal access) with lower risk. Similarly, a financial AI trained on decades of biased credit decisions could continue underserving minority applicants—not through intentional discrimination but by mirroring biases in its input data.
Sometimes bias emerges not just from data but from how models operate on that data. AI systems latch onto any correlations that help optimize their objective—even ethically problematic ones. Even if we remove explicit indicators of race or gender from a loan approval model, the AI might still infer those attributes through proxies (like neighborhood or employment history), thereby reproducing bias.
Many AI models tune to maximize overall accuracy or profit, which creates biased outcomes with imbalanced data. If one group overrepresents in training data, the model becomes very accurate for that majority group, treating it as the "norm" at the expense of minorities.
Practical Solutions to Prevent AI Hallucinations
Fortunately, there are effective approaches to reduce hallucinations in financial AI systems:
1. Domain-Specific Fine-Tuning
One root cause of hallucinations is that general-purpose models lack specialized financial knowledge. By fine-tuning an AI model on relevant, high-quality financial data, you can significantly improve its accuracy in that domain. Targeted training with historical financial reports, regulatory documents, and finance terminology helps the AI learn correct information rather than guessing.
As experts note, "training a model on more relevant and accurate information makes it more accurate at generating responses, thereby minimizing chances of hallucination." A bank might retrain an LLM using its internal knowledge base of approved investment research and current market data, reducing knowledge gaps and the likelihood of manufacturing answers about earnings or compliance rules.
2. Retrieval-Augmented Generation (RAG)
Retrieval-Augmented Generation grounds AI answers in factual reference material. With RAG, when the AI receives a query, it first searches a database for relevant documents, then bases its answer on that retrieved information. This means the model doesn't rely solely on its internal memory (which might be outdated); instead, it's "augmented" with a live source of truth.
For example, if asked about stock performance, a RAG-based assistant would fetch the latest price from a trusted database and incorporate those facts into its reply, rather than inventing numbers. By connecting generation to real data, RAG dramatically reduces hallucination rates and increases factual accuracy.
3. Advanced Prompting Techniques
How you prompt an LLM significantly influences whether it strays from facts. Advanced methods like chain-of-thought prompting encourage models to reason step-by-step, reducing mistakes. In chain-of-thought, the prompt guides the AI to break down problems and show reasoning, which catches contradictions before they appear in final answers.
Similarly, few-shot prompting (providing examples of correct Q&A in the prompt) sets standards for expected answers. These techniques make AI more likely to remain factual. For instance, a chain-of-thought prompt for financial questions might lead models to calculate figures stepwise rather than guessing arbitrary numbers.
4. AI Guardrails and Verification
Guardrails are external checks and rules imposed on AI systems to catch undesired outputs. They might include validation routines, filters, or business rules that intercept responses before reaching end-users. In finance, a guardrail might verify numerical outputs against known ranges or reconcile with source data.
For example, if the AI claims "Company X had a net profit of $500 million in 2024," a guardrail could compare against official reported earnings and flag discrepancies. Modern guardrail frameworks can enforce source citations for factual claims and check sources for consistency.
5. Consensus and Cross-Verification
An emerging technique uses multiple AI models (or multiple prompts) in parallel to compare results. While one model might hallucinate, it's unlikely several models will hallucinate identical false answers. In a recent industry experiment, financial firms and technology providers used a "swarm" of LLMs to parse corporate action documents and convert them to structured formats.
They found that requiring consensus—only accepting outputs when multiple models agreed—greatly reduced hallucination risks. When all models produced identical interpretations, results were deemed trustworthy; disagreements flagged potential errors for manual review.
6. Continuous Monitoring and Improvement
Mitigating hallucinations isn't a one-time setup but an ongoing process. Organizations should establish continuous monitoring of AI outputs, user feedback loops, and periodic performance evaluations. If certain questions consistently trigger hallucinations, they should be identified and used to retrain models.
Techniques like Decoding by Contrasting Layers (DoLa) and other algorithms are developing to reduce hallucination tendencies at the decoding stage. Staying current with latest improvements and regularly updating models gradually reduces hallucination problems.
The Path Forward
AI and large language models offer transformative opportunities for financial businesses—from automating customer support to analyzing market data—but they come with the critical challenge of maintaining truthfulness and fairness. AI hallucinations pose tangible risks in finance, including faulty decisions, compliance breaches, and trust erosion.
Organizations should approach AI deployment with both caution and control: robust data governance, bias mitigation, and technical solutions (like RAG, fine-tuning, and guardrails) are essential components of responsible AI strategy. Financial institutions are already implementing frameworks to overcome these issues—using retrieval techniques to ground model answers in real data and instituting human oversight for AI outputs.
Encouragingly, ongoing research continues improving our ability to detect and reduce hallucinations, while regulators provide guidance on ethical AI use in finance. With proper guardrails, AI systems can greatly enhance financial decision-making and efficiency—but we must ensure these systems "hallucinate" as little as possible and never at the expense of integrity or compliance.
The future of financial AI requires careful innovation: embracing new technologies while maintaining firm control over risks and committing to ethical, reliable outcomes.
At Baytech Consulting, we specialize in guiding businesses through this process, helping you build scalable, efficient, and high-performing software that evolves with your needs. Our MVP first approach helps our clients minimize upfront costs and maximize ROI. Ready to take the next step in your software development journey? Contact us today to learn how we can help you achieve your goals with a phased development approach.
About the Author
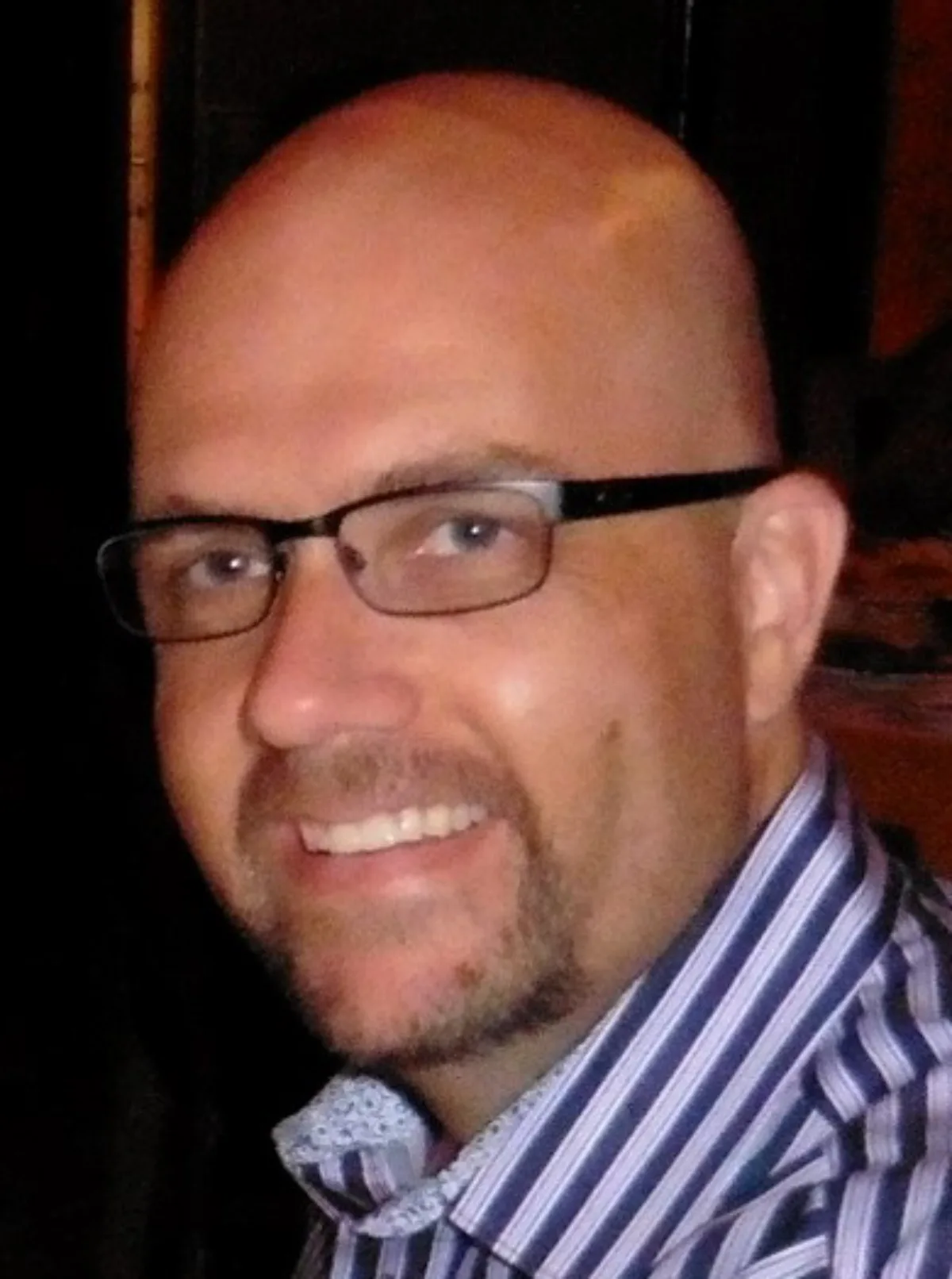
Bryan Reynolds is an accomplished technology executive with more than 25 years of experience leading innovation in the software industry. As the CEO and founder of Baytech Consulting, he has built a reputation for delivering custom software solutions that help businesses streamline operations, enhance customer experiences, and drive growth.
Bryan’s expertise spans custom software development, cloud infrastructure, artificial intelligence, and strategic business consulting, making him a trusted advisor and thought leader across a wide range of industries.