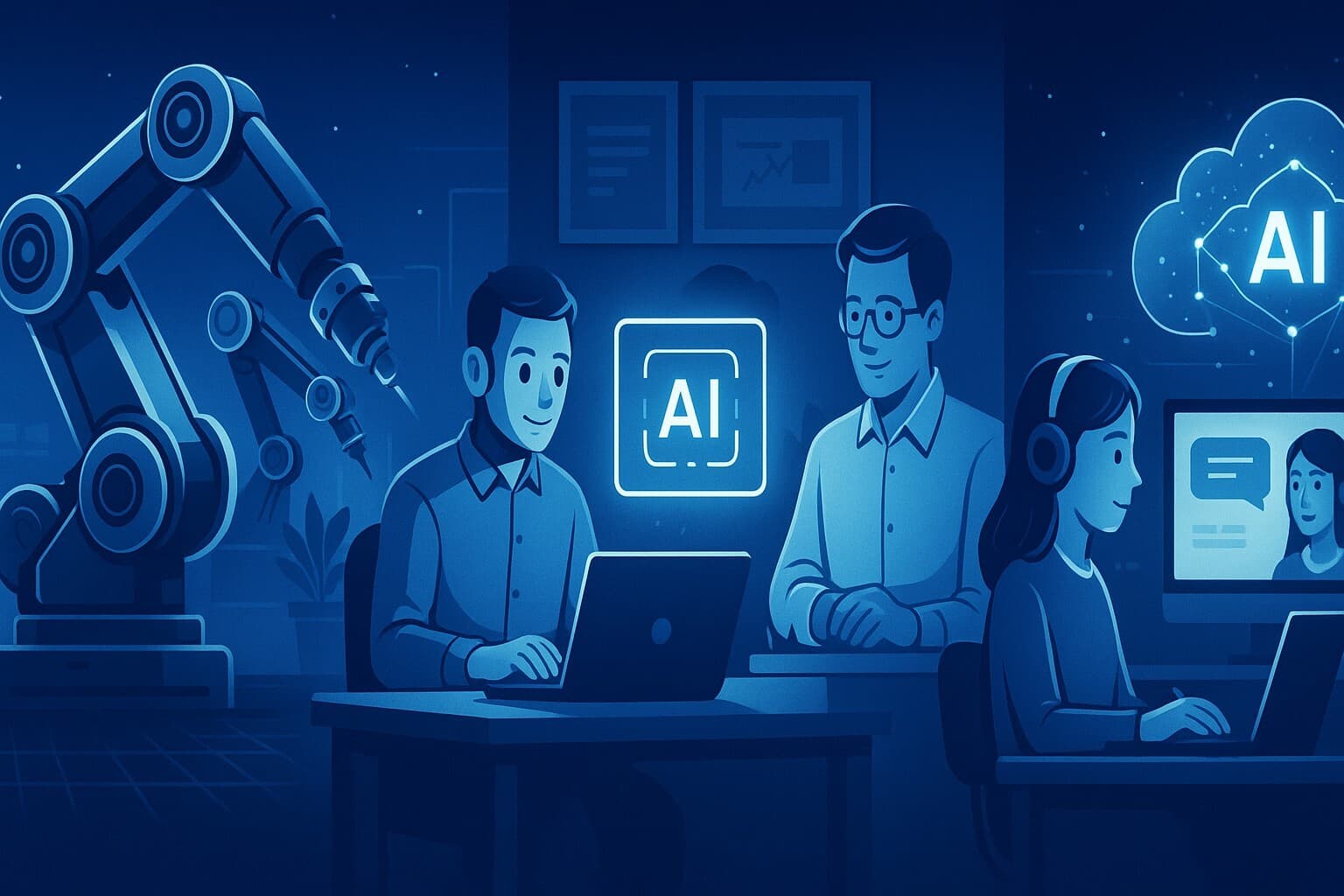
The State of Artificial Intelligence in 2025: Acceleration, Economics, and Ecosystem Dynamics
May 27, 2025 / Bryan ReynoldsThe year 2025 marks a significant inflection point for Artificial Intelligence (AI). Moving decisively beyond the initial waves of generative AI hype, the field is now characterized by tangible integration into the fabric of society and the economy. This period is defined by several converging themes: remarkable acceleration in AI performance coupled with significant efficiency gains, making powerful models more accessible ; unprecedented corporate adoption fueled by record levels of investment, particularly in generative AI ; a dynamic and increasingly competitive interplay between rapidly advancing open-source models and established proprietary systems ; a growing understanding of the complex, often bifurcated economics governing AI development, training, and deployment ; the emergence and refinement of transformative capabilities like agentic and multimodal AI, promising new paradigms of interaction and automation ; and an intensifying focus on the critical need for responsible AI development, robust governance frameworks, and effective risk mitigation strategies to address the technology's potential downsides. This report provides a comprehensive, data-driven guide to this rapidly evolving landscape, analyzing the key technological advancements, economic factors, market players, and trending applications shaping the state of AI in 2025.
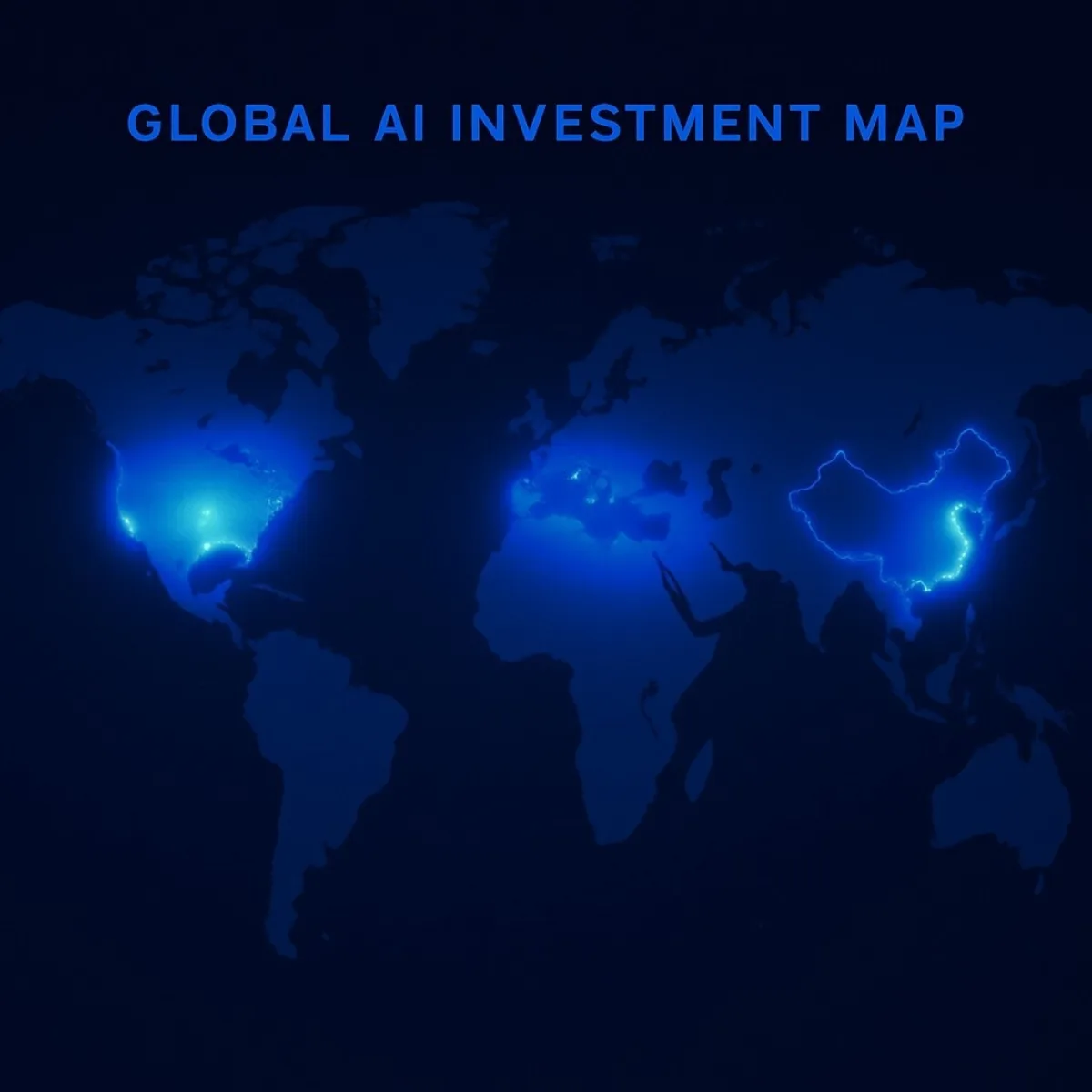
Section 1: The State of AI in 2025: Performance, Efficiency, and Adoption
The AI landscape in 2025 is defined by relentless progress on multiple fronts. Foundational models continue to achieve new levels of performance on increasingly difficult tasks, while simultaneous breakthroughs in efficiency are making these powerful capabilities more affordable and accessible than ever before. This confluence of factors is driving a surge in corporate adoption and investment, embedding AI more deeply into business operations and daily life.
1.1 Benchmarking the Frontier: AI Performance Leaps
The capabilities of AI systems continue their steep upward trajectory, evidenced by significant gains on standardized benchmarks designed to push the limits of machine intelligence. In 2023, researchers introduced several new, demanding benchmarks, including MMMU (Massive Multi-discipline Multimodal Understanding), GPQA (Graduate-Level Google-Proof Q&A), and SWE-bench (Software Engineering Benchmark), to rigorously test advanced AI systems. Within just one year, performance on these challenging tests increased dramatically. Scores rose by 18.8 percentage points on MMMU, 48.9 percentage points on GPQA, and a remarkable 67.3 percentage points on SWE-bench. This rapid improvement across diverse tasks-spanning multimodal understanding, complex reasoning, and code generation-underscores the continued potency of current AI architectures and training methodologies. While discussions about potential performance plateaus exist , the empirical evidence from 2024-2025 points towards substantial remaining headroom for capability growth.
The competitive landscape at the AI frontier is also intensifying, with performance gaps between leading models narrowing considerably. According to the AI Index 2025 report, the Elo skill score difference (a measure used to rank players in competitive games, adapted here for AI models) between the top-ranked and 10th-ranked AI models decreased from 11.9% to just 5.4% over the course of a year. Furthermore, the gap between the top two models shrank to a mere 0.7%. This convergence suggests that multiple research labs and organizations are achieving state-of-the-art results, making it increasingly difficult for any single entity to maintain a dominant performance lead based solely on benchmark scores. The frontier is becoming both more capable and more crowded.
Beyond standardized tests, AI systems are demonstrating significant progress in complex generative tasks and specific applications. Major strides have been made in generating high-quality, coherent video content. In certain contexts, particularly those with tight time constraints, AI agents have even outperformed human experts in programming tasks. However, it's crucial to note the nuances: when tasks allow for extended time and deeper deliberation (e.g., 32 hours vs. 2 hours), human experts still tend to outperform current AI systems, often by a significant margin (2-to-1). This highlights that while AI excels in speed, pattern matching, and executing known procedures, human strengths in deep, flexible reasoning, common-sense understanding, and adaptability over extended, complex problems remain distinct.
Table 1: AI Benchmark Performance Highlights (2024-2025)
Benchmark Category | Benchmark Example | Key Finding |
---|---|---|
Demanding Benchmarks | MMMU | Score increase of 18.8 percentage points in one year (2024-2025) |
GPQA | Score increase of 48.9 percentage points in one year (2024-2025) | |
SWE-bench | Score increase of 67.3 percentage points in one year (2024-2025) | |
Model Competition | Elo Skill Scores | Gap between top and 10th model decreased from 11.9% to 5.4% in one year; Gap between top two models is 0.7% |
Cross-National Progress | MMLU, HumanEval | Performance gap between US and Chinese models shrank from double digits (2023) to near parity (2024) |
Human vs. AI (Time) | Complex Tasks | AI outperforms humans 4x in short (2hr) settings; Humans outperform AI 2x when given more time (32hr) |
1.2 More for Less: Efficiency Gains and Cost Reductions in AI Models
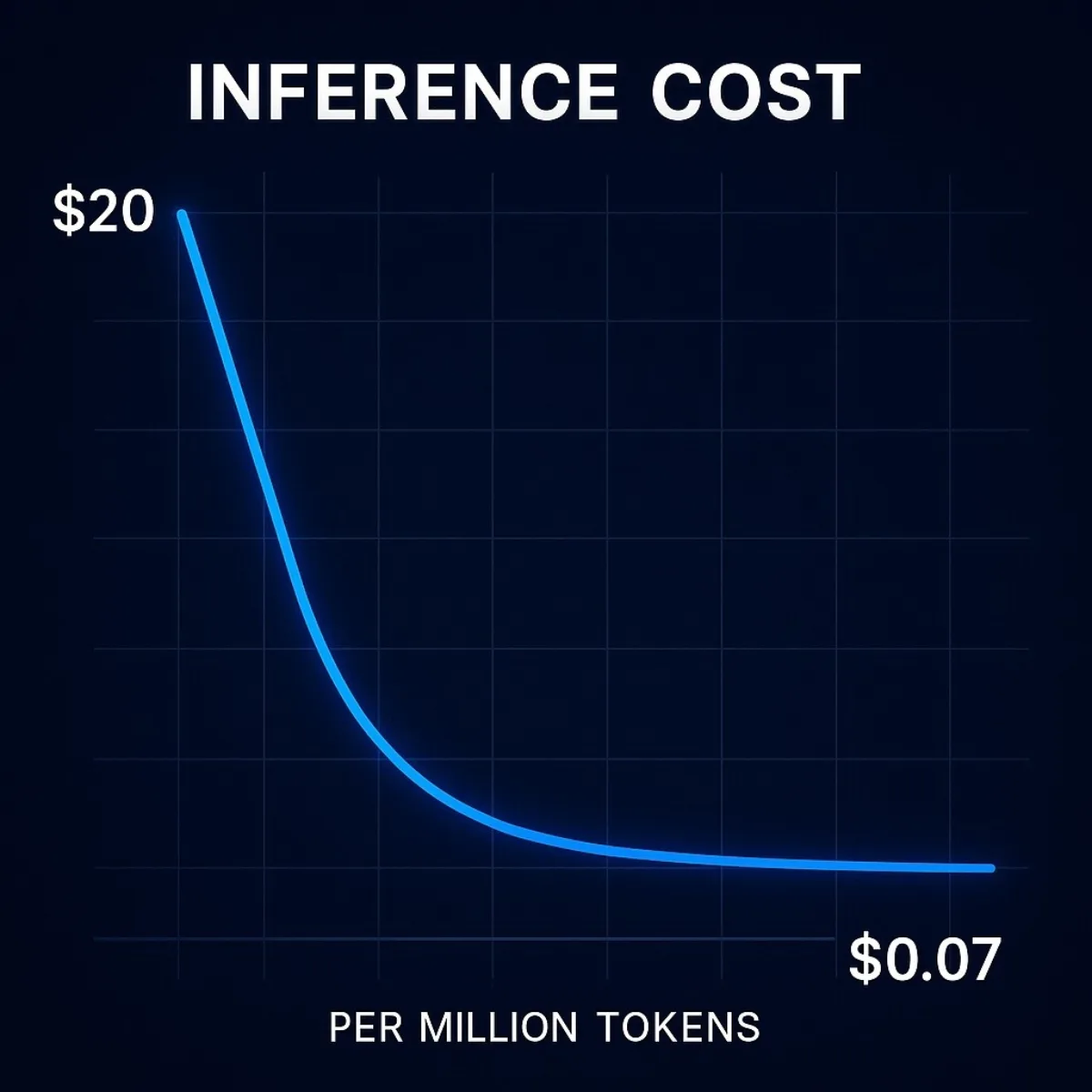
Parallel to performance improvements, a critical trend shaping the AI landscape in 2025 is the dramatic increase in model efficiency and the corresponding reduction in deployment costs. One of the most significant developments is the rise of highly capable Small Language Models (SLMs). These models pack impressive performance into a much smaller parameter count compared to their massive predecessors. A striking example comes from the MMLU benchmark: in 2022, achieving a score above 60% required a model like Google's PaLM, boasting 540 billion parameters. By 2024, Microsoft's Phi-3-mini achieved the same performance threshold with just 3.8 billion parameters-a staggering 142-fold reduction in model size in just over two years. This trend towards smaller, yet powerful, models is crucial. It enables advanced AI capabilities to run on less powerful hardware, including edge devices like smartphones and laptops , significantly reducing energy consumption and broadening the accessibility of sophisticated AI beyond large data centers.
Perhaps even more impactful for widespread adoption is the plummeting cost of using trained AI models, known as inference cost. The expense associated with querying an AI model to get a response has fallen precipitously. Using the performance level of GPT-3.5 on the MMLU benchmark (64.8% accuracy) as a reference, the cost dropped from $20 per million tokens in November 2022 to a mere $0.07 per million tokens by October 2024 when using a model like Google's Gemini-1.5-Flash-8B. This represents an over 280-fold cost reduction in approximately 18 months. Stanford's AI Index report further notes that depending on the specific task, LLM inference prices have decreased anywhere from 9 to 900 times per year. This drastic reduction makes it economically feasible for a much wider array of businesses and developers to experiment with, integrate, and deploy AI solutions at scale, transforming AI from a niche, expensive technology into a more accessible utility.
These model-level improvements are complemented by favorable trends in the underlying hardware. Hardware costs for AI computation have been declining at an estimated rate of 30% annually, while the energy efficiency of this hardware has been improving by approximately 40% each year. Together, more efficient models, cheaper inference pricing, and improving hardware create a powerful cycle that lowers the barrier to entry for AI deployment.
This combination of plummeting inference costs , increasingly capable smaller models , and the improving performance of open-source alternatives points towards a significant democratization of AI deployment. More organizations than ever can leverage sophisticated AI capabilities. However, this trend exists in tension with the economics of creating the next generation of frontier models. Training these state-of-the-art systems requires immense capital for compute power, vast datasets, and specialized talent, with costs running into the hundreds of millions of dollars. This concentrates the power to push the absolute boundaries of AI research within a small number of large, well-funded technology companies and research labs. Consequently, the AI ecosystem in 2025 exhibits a dynamic where the ability to use and apply AI is becoming more distributed and accessible, while the capacity to build the most advanced foundational models remains highly centralized. This suggests a future where value creation may increasingly shift towards innovative applications, efficient deployment, and specialized fine-tuning built upon foundational models provided by a few key players, rather than solely on access to raw model capabilities.
Table 2: AI Inference Cost Trends (Selected Models/Tasks, 2022-2025)
Model/Task Equivalent | Time Period | Cost per Million Tokens (Input/Output where applicable) | Fold Reduction (Approx.) |
---|---|---|---|
GPT-3.5 Level (MMLU 64.8%) | Nov 2022 | $20 | - |
GPT-3.5 Level (MMLU 64.8%) | Oct 2024 (Gemini-1.5-Flash-8B) | $0.07 | >280x |
GPT-4o (OpenAI) | 2025 Pricing | ~$5 (Input) / ~$15 (Output) | N/A |
Claude 3 Sonnet (Anthropic) | 2025 Pricing | ~$3 (Input, includes output) | N/A |
Gemini 2 Pro (Google) | 2025 Pricing | ~$3-$5 (Input, includes output) | N/A |
DeepSeek V3 | 2025 Pricing | ~$0.50-$1.50 (Input, includes output) | N/A |
OpenAI Embedding (text-embedding-3-small) | 2025 Pricing | ~$0.10 | N/A |
General LLM Inference | Annual Trend | 9x to 900x decrease per year (task dependent) | N/A |
1.3 AI Integration: Corporate Adoption and Investment Surge
Fueled by demonstrable performance gains and falling deployment costs, the integration of AI into the corporate world has shifted into high gear in 2025. Enterprise adoption rates have accelerated dramatically. According to survey data cited in the AI Index 2025 report and McKinsey studies, 78% of organizations reported using AI in at least one business function in 2024, a substantial jump from 55% in 2023. The adoption of generative AI, specifically, has seen explosive growth, with the number of organizations reporting its use in at least one business function more than doubling from 33% in 2023 to 71% in 2024. This rapid uptake signifies that AI is transitioning from an experimental technology explored by early adopters to a tool being actively integrated into core business operations across diverse departments, including customer support, marketing, IT, operations, and R&D.
This adoption wave is underpinned by record levels of private investment flowing into the AI sector. The United States, in particular, saw a surge in private AI investment, reaching $109.1 billion in 2024. This figure dwarfs investments in other leading nations, being nearly 12 times higher than China's $9.3 billion and 24 times higher than the UK's $4.5 billion. Generative AI has been a major focus of this investment boom, attracting $33.9 billion globally in private investment in 2024, an 18.7% increase from the previous year. The number of newly funded generative AI startups also nearly tripled, indicating strong investor confidence in the technology's disruptive potential and economic prospects. This influx of capital fuels further research, development of new tools and platforms, and talent acquisition, creating a positive feedback loop that further accelerates AI adoption. The pronounced lead of the US in AI investment suggests it will likely remain the primary hub for major commercial AI development and deployment in the near future.
However, simply adopting AI tools is proving insufficient for unlocking deep, transformative value. Businesses are realizing that capturing significant bottom-line impact requires more than just plugging AI into existing structures. McKinsey's research emphasizes that realizing tangible benefits, measured by impact on Earnings Before Interest and Taxes (EBIT), is strongly correlated with the strategic redesign of workflows to leverage AI capabilities. While adoption is widespread, only 21% of organizations using generative AI reported having fundamentally redesigned at least some workflows in 2024. This indicates a maturing approach where AI acts not merely as an add-on tool but as a catalyst for fundamental process transformation. Such transformation is deemed essential for capturing the substantial productivity gains promised by AI, as confirmed by a growing body of research showing AI's positive impact on productivity and its potential to narrow skill gaps. Furthermore, strong senior leadership involvement, particularly CEO oversight of AI governance, is also correlated with achieving higher bottom-line impact from AI initiatives.
The gap between the high rate of AI adoption (78%) and the much lower rate of fundamental workflow redesign (21%) suggests that many organizations are still in the early phases of their AI journey. They may be achieving localized efficiencies or automating specific tasks, but the deeper, systemic value that comes from rethinking core processes remains largely untapped for the majority. This points to a critical imperative for businesses in 2025 and beyond: moving beyond superficial adoption towards deep, strategic integration and process transformation. Companies that successfully navigate this complex transition-likely those with strong leadership commitment, a clear vision for AI integration, and a willingness to invest in change management and workflow redesign-are poised to gain a significant competitive advantage by fully realizing AI's productivity potential.
Beyond the corporate sphere, AI's integration into daily life is becoming increasingly evident. The U.S. Food and Drug Administration (FDA) has seen a dramatic increase in approvals for AI-enabled medical devices. After approving its first such device in 1995, only six had been authorized by 2015. This number skyrocketed to 223 cumulative approvals by the end of 2023. In transportation, self-driving car services are moving beyond experimentation; Waymo, a major US operator, reported providing over 150,000 autonomous rides each week, while Baidu's Apollo Go robotaxi service continues its expansion across numerous cities in China. These examples illustrate AI's tangible impact on consumers and society, moving from research labs into real-world applications.
1.4 The Global AI Landscape: Competition and Collaboration
The development and deployment of AI in 2025 is occurring within a complex global context marked by intense competition, primarily between the United States and China, but also featuring growing contributions from other regions. The US currently maintains a lead in producing the highest number of notable, frontier AI models, with US-based institutions responsible for 40 such models in 2024, compared to 15 from China and only three from Europe. This lead in cutting-edge model development is mirrored by the nation's dominance in private AI investment, as detailed previously. This advantage is sustained by a robust ecosystem encompassing leading universities, abundant venture capital, and the headquarters of most major global technology firms.
However, while the US leads in quantity of top models and funding, China is rapidly closing the gap in terms of model quality and performance. Performance differences between US and Chinese models on major benchmarks like MMLU and HumanEval, which were in the double digits in 2023, narrowed significantly to near parity by 2024. Furthermore, China continues to lead the world in the sheer volume of AI-related publications and patent filings. This indicates that Chinese researchers and institutions are quickly catching up in technical capabilities and are highly active in foundational AI research, contributing to an increasingly competitive global dynamic.
The AI landscape is not solely defined by the US-China rivalry. Model development is becoming increasingly globalized, with notable contributions and model launches emerging from regions such as the Middle East, Latin America, and Southeast Asia. The proliferation of open-source models and frameworks plays a significant role here, lowering barriers to entry and enabling broader participation in AI development and adaptation worldwide. This diversification enriches the global AI ecosystem and fosters innovation beyond the traditional power centers.
Public perception of AI and its potential impact also varies considerably across the globe, potentially influencing adoption rates and regulatory postures. Surveys conducted in 2024 revealed high levels of optimism in several Asian countries, with strong majorities in China (83%), Indonesia (80%), and Thailand (77%) believing that AI products and services offer more benefits than drawbacks. In contrast, optimism remains significantly lower in many Western nations, including Canada (40%), the United States (39%), and the Netherlands (36%). However, a noteworthy shift has occurred since 2022, with optimism showing significant growth in several previously more skeptical Western countries like Germany (+10%), France (+10%), Canada (+8%), Great Britain (+8%), and the United States (+4%). These differing attitudes likely reflect a combination of cultural contexts, government narratives regarding technology, direct experiences with AI tools, and media coverage. The rising optimism in some Western countries might be linked to the increased visibility and perceived utility of widely accessible generative AI tools like ChatGPT.
1.5 Navigating the Nuances: Responsible AI, Risks, and Regulation
The rapid advancement and proliferation of AI technologies in 2025 are accompanied by growing concerns about risks, ethical implications, and the need for effective governance. As AI systems become more powerful and integrated into critical societal functions, the potential for misuse, unintended consequences, and societal harm increases. This is reflected in the sharp rise of documented AI-related incidents. The AI Incidents Database, which tracks failures and harms caused by AI systems, recorded 233 incidents in 2024, marking a significant 56.4% increase compared to 2023 and reaching a record high. Notable examples cited include the proliferation of deepfake intimate images and instances where chatbots were allegedly implicated in harmful real-world events. The rapid improvement and accessibility of technologies like deepfakes, which saw a tenfold global surge in 2023 , underscore the urgency of addressing these risks.
Despite the clear rise in incidents and growing awareness of potential harms, the development and adoption of robust Responsible AI (RAI) practices within the industry appear to be lagging behind technological capabilities. Standardized evaluations for RAI principles like fairness, transparency, and safety remain rare among major industrial AI model developers. Surveys suggest a persistent gap between companies acknowledging RAI risks and implementing meaningful, concrete actions to mitigate them. However, progress is being made in developing tools and benchmarks to better assess these aspects, with examples like HELM Safety, AIR-Bench, and FACTS offering promising methods for evaluating model factuality and safety. Within organizations, there is an increasing effort to manage specific generative AI risks, particularly inaccuracy, cybersecurity vulnerabilities, and potential intellectual property infringement. Nearly half (47%) of organizations reported experiencing negative consequences related to these risks in 2024. Yet, oversight practices vary widely; for instance, only 27% of organizations using generative AI reported reviewing all AI-generated content before external use , suggesting a lack of consistent internal governance standards. Privacy concerns are also paramount, driven by AI's reliance on vast amounts of data.
In contrast to the uneven progress within industry, governments globally are demonstrating increased urgency in addressing AI governance. International cooperation intensified in 2024, with major intergovernmental organizations including the OECD, European Union, United Nations, and the African Union releasing AI governance frameworks. These frameworks commonly focus on core principles such as transparency, trustworthiness, accountability, and fairness. Regulatory activity is also accelerating at the national and sub-national levels. In the United States, while progress on comprehensive federal AI legislation remains slow, state-level AI-related laws are surging. In 2023, 49 such laws were passed, and in the past year alone (leading into 2025), that number more than doubled to 131.
This situation highlights a significant "governance gap": the pace of AI technological advancement and its rapid deployment are outstripping the development and implementation of effective mechanisms to manage its risks and ensure responsible use. Both corporate practices and comprehensive regulatory frameworks are struggling to keep pace. Developing robust safety protocols, ethical guidelines, standardized evaluation methods, and effective legal frameworks is inherently a slower, more complex process than iterating on technology. It requires careful deliberation, consensus-building, empirical testing, and adaptation. The surge in state-level legislation in the US, while reactive to the perceived need for action, risks creating a fragmented and complex regulatory landscape that could potentially stifle innovation or impose significant compliance burdens on businesses operating across multiple jurisdictions. Navigating this governance gap represents a critical challenge for the AI ecosystem in 2025. Companies face the dual pressures of innovating rapidly to remain competitive while simultaneously managing poorly understood risks and anticipating an evolving, potentially patchwork regulatory environment. Achieving a sustainable balance between fostering innovation and ensuring responsible, ethical deployment is paramount, making the development and adoption of effective RAI tools, frameworks, and practices increasingly critical.
Section 2: The Open Source AI Ecosystem: Acceleration Through Collaboration
Alongside the advancements driven by large technology companies and well-funded startups, the open-source AI ecosystem has become a powerful force in 2025, significantly shaping the trajectory of AI development and deployment through collaborative innovation.
2.1 Leading Open Source Models and Their Capabilities
A vibrant and rapidly evolving landscape of powerful open-source or "open-weight" (models whose weights are released publicly, though training data or code might not be fully open) AI models has emerged, offering compelling alternatives to proprietary systems. These models are developed and shared by a mix of academic institutions, startups, and even major technology companies seeking to foster community engagement or establish alternative standards.
Key players and model families driving open-source AI in 2025 include:
- Meta's Llama Series: Meta has positioned itself as a major champion of open source with its Llama models. Llama 2 gained widespread adoption, and the Llama 3 series (including 8B and 70B parameter versions, with larger models planned) represents a significant step up in capability, trained on vast datasets and demonstrating strong performance in reasoning and instruction following.
- Mistral AI's Models: This European startup has made waves with its highly efficient and performant models. Mistral 7B offered strong capabilities in a compact size, while the Mixtral series (e.g., Mixtral 8x7B, Mixtral 8x22B) utilizes a Sparse Mixture-of-Experts (SMoE) architecture to deliver high performance with greater computational efficiency during inference compared to dense models of similar capability.
- Microsoft's Phi Series: While Microsoft heavily invests in proprietary AI via OpenAI and Azure, it has also contributed significantly to the open-source space with its Phi models (Phi-1, Phi-2, Phi-3, Phi-4). These models are notable for achieving remarkable performance, particularly in reasoning and coding tasks, within relatively small parameter counts (e.g., Phi-3-mini at 3.8B parameters), making them suitable for on-device or resource-constrained deployments.
- DeepSeek Models: This Chinese AI startup has released models like DeepSeek-Coder and DeepSeek V2/V3, aiming for strong performance, particularly in coding and multilingual tasks, often coupled with disruptive API pricing for their hosted versions, challenging established players.
- Other Notable Models: The ecosystem includes contributions from various other players, such as Google's Gemma models (derived from Gemini technology but released openly), models from EleutherAI, TII (Falcon), and numerous specialized models available on platforms like Hugging Face.
Crucially, the performance gap between leading open-weight models and their closed-source, proprietary counterparts is narrowing rapidly. Stanford's AI Index 2025 reported that this gap diminished significantly over the past year, shrinking from an 8% difference to just 1.7% on certain benchmarks. This rapid convergence demonstrates the power and effectiveness of the open development model, where community contributions, shared research, and rapid iteration accelerate progress. This trend puts competitive pressure on proprietary model providers and offers users increasingly viable and powerful open alternatives. The diversity within the open-source landscape allows developers and organizations to select models that best fit their specific requirements regarding performance trade-offs, model size, computational budget, licensing constraints, and task specialization (e.g., coding, reasoning, multilingual capabilities).
Table 3: Comparison of Leading Open Source / Open-Weight Models (2025)
Model Family | Developer | Key Features | Notable Performance / Use Cases | Licensing Notes (General) |
---|---|---|---|---|
Llama 3 | Meta | 8B, 70B params (400B+ planned); 15T token dataset; 128k vocab; 8k context; GQA; Open Weights | Strong reasoning, coding, instruction following; Versatile (content gen, chat, analysis) | Custom Llama 3 License |
Mistral / Mixtral | Mistral AI | Mistral 7B (compact); Mixtral 8x7B, 8x22B (SMoE architecture, efficient inference); Open Weights | High performance/efficiency ratio; Multilingual; Strong math/coding (Mixtral 8x22B: 64k context) | Apache 2.0 (for base models) |
Phi-4 Series | Microsoft | Phi-4-mini (3.8B), Phi-4 (14B); Phi-4-reasoning variants; Phi-4-multimodal (5.6B); Open Weights (check license) | High capability SLMs; Strong reasoning/math/coding; Multimodal input; Function calling; Edge deploy | MIT License (typically) |
DeepSeek V3 | DeepSeek AI | 67B params (MoE?); Focus on coding & multilingual; Open Weights | Competitive performance; Low-cost API alternative; Good for low-resource languages | Custom DeepSeek License |
Gemma | 2B, 7B params; Derived from Gemini; Open Weights | Solid general capabilities; Good starting point for fine-tuning | Custom Gemma License |
(Note: Licensing terms can be complex and specific to model versions; users should always verify the license for their intended use case. Performance varies significantly based on fine-tuning and evaluation methods.)
2.2 Foundational Frameworks: Building Blocks of Open AI Development
The rapid development and deployment of AI applications, both open and closed source, rely heavily on a rich ecosystem of open-source software frameworks and libraries. These tools provide the essential building blocks, abstractions, and utilities that researchers and developers use to create, train, evaluate, and deploy AI models.
At the foundational level are the core deep learning libraries:
- TensorFlow: Originally developed by Google Brain, TensorFlow remains a comprehensive ecosystem for machine learning development, supporting everything from research prototyping to large-scale production deployment. It features robust neural network capabilities and strong support within the Google ecosystem.
- PyTorch: Created by Meta's AI Research lab (FAIR), PyTorch has gained immense popularity, especially within the research community, due to its dynamic computational graph (allowing for more flexible model definition and debugging), Pythonic interface, and strong GPU acceleration support.
- Keras: Often used as a high-level API that can run on top of backends like TensorFlow, Keras simplifies the process of building and experimenting with neural networks, making it particularly suitable for beginners and rapid prototyping.
- Apache MXNet: Known for its scalability and efficiency, particularly in distributed training scenarios across multiple GPUs or machines, MXNet is favored for large-scale enterprise projects.
Building upon these core libraries, a layer of higher-level frameworks has emerged to address specific needs in the era of large language models (LLMs) and generative AI:
- Hugging Face Transformers: This library has become a de facto standard in Natural Language Processing (NLP). It provides access to thousands of pre-trained models (including many leading open-source LLMs), along with tools for easy downloading, fine-tuning, and deployment. The associated Hugging Face Hub serves as a central repository for models, datasets, and demos, fostering a massive collaborative community.
- LangChain: A highly popular framework for developing applications powered by LLMs. LangChain simplifies the process of building complex workflows by providing modular components ("chains") for connecting LLMs to data sources, APIs, memory systems, and other tools. It's widely used for creating chatbots, question-answering systems, summarization tools, and agentic applications.
- LlamaIndex: Specifically designed as a data framework to connect LLMs with external, often private, data sources. It focuses on efficient data ingestion, indexing (creating vector embeddings), and retrieval, making it a cornerstone for building Retrieval-Augmented Generation (RAG) applications, where LLMs access external knowledge to provide more accurate and context-aware responses.
- AI Agent Frameworks: As interest in agentic AI grows, frameworks are emerging to facilitate their development. Examples include Microsoft's AutoGen (focused on automating multi-agent conversations and workflows) , CrewAI (for orchestrating role-playing AI agents) , and LangGraph (an extension of LangChain for building stateful, multi-actor applications).
- RAG Frameworks: Beyond LlamaIndex, numerous frameworks specialize in building RAG pipelines, each with different strengths, such as Dify (visual development), RAGFlow (deep document understanding), Haystack (production pipelines), and Milvus (scalable vector search).
These frameworks play a crucial role in the AI ecosystem. They abstract away significant low-level complexity, allowing developers to focus on application logic and innovation rather than reinventing fundamental components. They promote standardization and best practices, facilitating collaboration and knowledge sharing. By making it easier to leverage powerful open-source models, these frameworks accelerate the development and deployment of sophisticated AI applications, contributing significantly to the dynamism and accessibility of the field.
2.3 The Open Advantage: Adaptability, Cost-Efficiency, and Privacy
The growing adoption of open-source AI models and frameworks is driven by several key advantages they offer compared to proprietary, closed-source alternatives, particularly for enterprises and developers seeking greater control and flexibility.
- Adaptability and Customization: Perhaps the most significant advantage is the ability to modify and tailor open models to specific needs. Enterprises can fine-tune pre-trained open models like Llama 3 or Mistral 7B on their own proprietary data to specialize them for particular tasks (e.g., understanding industry-specific jargon, detecting specific types of financial fraud, adopting a particular brand voice for customer service). Frameworks like LoRA (Low-Rank Adaptation) and PEFT (Parameter-Efficient Fine-Tuning) make this customization process more computationally feasible. This level of deep customization often goes beyond what is possible with generic, black-box proprietary APIs, allowing for potentially higher performance on niche tasks.
- Cost Efficiency: Open-source models offer substantial cost benefits. Firstly, they eliminate the direct per-token or per-API-call costs associated with using proprietary models hosted by vendors. While running open-source models still incurs compute costs (for hosting and inference), these can be significantly lower, especially at scale or when using highly efficient models. Models like Microsoft's Phi series or Mistral 7B are designed for efficiency and can run on less powerful, cheaper hardware, potentially reducing cloud inference costs by up to 40% compared to larger models. This efficiency also enables deployment on edge devices (IoT, mobile), reducing reliance on cloud infrastructure.
- Privacy and Security: For organizations handling sensitive data (e.g., healthcare, finance, customer PII), the ability to self-host open-source models within their own infrastructure is a major draw. This ensures that confidential data never leaves the organization's control, mitigating risks associated with sending data to third-party API providers and facilitating compliance with stringent data privacy regulations like GDPR (General Data Protection Regulation) in Europe or HIPAA (Health Insurance Portability and Accountability Act) in the US.
- Transparency and Collaboration: Open source inherently promotes transparency. While the degree of openness varies (model weights vs. full training data/code), it generally provides greater visibility into model architecture and potentially training data compared to opaque proprietary systems. This transparency can aid in understanding model behavior, identifying potential biases, and fostering trust. Furthermore, the open nature allows a global community of researchers and developers to inspect, critique, improve, and build upon the models, accelerating innovation and bug fixing through collective effort. Platforms like Hugging Face act as central hubs for this collaborative ecosystem.
These combined advantages make open-source AI an increasingly compelling proposition in 2025, driving its adoption across various sectors for applications where customization, cost control, data sovereignty, and transparency are key priorities.
2.4 Open vs. Proprietary: The Evolving Dynamic
Despite the rapid rise and compelling advantages of open-source AI, the landscape in 2025 is characterized by a dynamic interplay and ongoing competition between open and proprietary approaches, rather than a complete shift towards one or the other.
While open-weight models are undeniably closing the performance gap and are projected to capture a significant share of enterprise AI deployments (estimated 25-30% by mid-2025) , leading proprietary models often maintain an edge at the absolute cutting edge of performance and capabilities. Models like OpenAI's latest GPT/o-series , Google's Gemini family , and Anthropic's Claude series frequently top leaderboards, particularly for complex reasoning, handling extremely long context windows, or advanced multimodal tasks. These companies invest hundreds of millions, potentially billions, in training runs , giving them an advantage in raw scale and access to the latest architectural innovations. Furthermore, proprietary models are often delivered via highly polished, easy-to-use APIs and platforms, offering convenience and potentially faster access to the very latest features for organizations prioritizing speed and simplicity over deep customization.
The strategic approaches of major players reflect this complex dynamic. Meta stands out as a strong proponent of open source with Llama, likely aiming to build a large ecosystem and challenge the dominance of closed API providers. Conversely, OpenAI and Anthropic primarily pursue a proprietary model, focusing on delivering state-of-the-art capabilities and safety through controlled development and API access. Google and Microsoft employ hybrid strategies; they develop and heavily promote their flagship proprietary models (Gemini, Copilot integrated with OpenAI models) and cloud platforms (Google Cloud AI, Azure AI), while also contributing to the open ecosystem through research publications, frameworks (TensorFlow), and the release of specific open models (Gemma, Phi series). These varying strategies reflect different calculations regarding market positioning, monetization potential, ecosystem control, and the perceived benefits of community engagement versus proprietary advantage.
Some analyses suggest that the current surge in open-source competitiveness might be challenged as proprietary labs continue to push boundaries with next-generation architectures and massive training investments, potentially re-establishing a wider performance gap for the most demanding enterprise use cases. However, the open-source community has proven remarkably adept at rapidly adopting and replicating innovations.
The AI landscape in 2025 is therefore likely to remain characterized by this coexistence and competition. The choice between open and proprietary solutions involves a complex trade-off analysis for users, balancing factors like absolute performance needs, cost sensitivity, customization requirements, data privacy concerns, required expertise, and the desired speed of access to novel features. This dynamic environment, fueled by innovation from both sides, ultimately benefits the field by accelerating progress and offering a wider range of options to users. The open-source movement acts as a powerful accelerator and equalizer, lowering barriers to entry, fostering global collaboration, and driving innovation from the ground up. While effectively leveraging open source still requires technical expertise and infrastructure investment , it provides a crucial pathway for broader participation and prevents the complete consolidation of AI power within a few proprietary players.
Section 3: The Economics of AI: Analyzing Costs Across the Lifecycle
Understanding the financial landscape of AI in 2025 requires dissecting costs across the entire lifecycle, from initial development and massive-scale training runs to ongoing deployment and operational expenses. The economics are often characterized by stark contrasts: astronomical costs for frontier model creation versus rapidly falling costs for utilizing existing models via inference.
3.1 AI Development Costs: From Concept to Deployment
The cost of developing a specific AI solution or application varies enormously depending on its complexity, scope, data requirements, and the chosen development approach. Estimates suggest a wide range:
- Simple AI Features or Proof-of-Concepts (PoCs): $10,000 - $50,000. These often involve basic automation, rule-based systems, or integrating pre-built APIs for initial validation.
- Minimum Viable Products (MVPs): $50,000 - $150,000+. MVPs, potentially using fine-tuned generative AI models, aim to test market viability with core features.
- Mid-Complexity Solutions: $60,000 - $250,000+. These might involve custom model development for tasks like predictive analytics or NLP pipelines.
- Advanced/Enterprise-Grade AI: $150,000 - $500,000+, potentially exceeding $1 million. These complex systems often involve deep learning, multi-modal capabilities, large-scale GenAI, or integration into critical business processes.
A common practice to manage these costs and associated risks is to break projects down into phases: PoC (2-10 weeks, $10k-$100k), Pilot/MVP (3-6 months, $50k-$150k+), and Full-Scale Implementation (6-12+ months, $100k-$500k+).
Costs are distributed across different development stages, with typical allocations being :
- Discovery & Planning: 5-15%
- Data Preparation & Feature Engineering: 15-30%
- Model Development & Training: 20-40%
- Integration & Deployment: 10-20%
- Testing & Quality Assurance: 10-15%
The significant portion allocated to data preparation (which can consume up to 30% or more of the entire project cost, ranging from $5k to $100k+) underscores the critical and often underestimated effort required to acquire, clean, label, and structure high-quality data suitable for AI training. Poor data quality invariably leads to higher training costs and suboptimal results.
Costs also vary significantly depending on the specific type of AI solution being built. Indicative ranges for 2025 include :
- AI Chatbots (Rule-based/NLP/GenAI): $10,000 - $50,000+
- Recommendation Engines: $50,000 - $150,000+
- Predictive Analytics Systems: $60,000 - $300,000+
- Computer Vision Systems: $80,000 - $400,000+
- Custom NLP Models: $100,000 - $300,000+
- Generative AI Solutions (Text, Image, Code): $50,000 (MVP) - $500,000+
Furthermore, industry-specific requirements, particularly around regulation, data sensitivity, and complexity, influence costs. For instance, AI projects in Healthcare ($300k-$600k+) and Finance ($300k-$800k+) often command higher budgets compared to Retail ($200k-$500k+) due to these factors.
3.2 The Price of Intelligence: Foundation Model Training Expenditures
While developing specific AI applications involves manageable, albeit significant, costs, the expense associated with training state-of-the-art, large-scale foundation models (like those powering ChatGPT or Gemini) is orders of magnitude higher, reaching into the tens and often hundreds of millions of dollars.
These staggering costs stem from a confluence of factors :
- Compute Infrastructure: Training requires massive clusters of specialized AI accelerators, primarily high-end GPUs (like NVIDIA's A100 or H100), often numbering in the thousands or tens of thousands, running continuously for weeks or months. Renting this cloud compute power is a primary cost driver.
- Energy Consumption: Powering and cooling these large data centers represents a substantial operational expense.
- Data: Acquiring, cleaning, filtering, and processing the petabyte-scale datasets needed to train these models is a massive undertaking.
- Engineering Talent: Highly specialized and sought-after AI researchers and engineers command premium salaries and equity compensation, which can constitute a significant portion of the total cost (e.g., estimated at 49% for Gemini Ultra).
- Time and Experimentation: Model training involves extensive experimentation, tuning, and multiple runs, further escalating compute and personnel costs.
Estimates for training specific major models illustrate the scale (costs often inflation-adjusted or based on compute estimations) :
- GPT-3 (175B params, 2020): $4.6M - $15M+
- GPT-4 (~1.7T+ params, 2023): ~$78M - $79M
- Google PaLM 2 (2023): ~$29M
- Google Gemini 1.0 Ultra (~1.5T params, 2023): ~$191M - $192M
- Meta Llama 2 (70B params, 2023): ~$3M+ (likely understates full R&D)
- Meta Llama 3.1 (405B params, 2024): ~$170M+
- Mistral Large (123B params, 2024): ~$41M
- xAI Grok-2 (2024): ~$107M
Table 4: Estimated Training Costs for Major Foundation Models
Model | Developer | Year | Parameters (Est.) | Training Cost (Est., Inflation-Adjusted) |
---|---|---|---|---|
GPT-3 | OpenAI | 2020 | 175 Billion | $4.6M - $15M+ |
GPT-4 | OpenAI | 2023 | 1.7 Trillion+ | $78M - $79M |
PaLM 2 | 2023 | ~540 Billion? | $29M | |
Gemini 1.0 Ultra | 2023 | ~1.5 Trillion | $191M - $192M | |
Llama 2 (70B) | Meta | 2023 | 70 Billion | $3M+ |
Llama 3.1 (405B) | Meta | 2024 | 405 Billion | $170M+ |
Mistral Large | Mistral AI | 2024 | 123 Billion | $41M |
Grok-2 | xAI | 2024 | Unknown | $107M |
Claude 3 Sonnet | Anthropic | 2024 | Unknown | Tens of Millions |
DeepSeek V3 | DeepSeek AI | 2024 | 67 Billion | $5.6M - $6M (Disputed) |
(Note: These figures are estimates based on available data and compute cost calculations; actual costs can vary and often include significant R&D personnel expenses not fully captured by compute estimates alone.)
The sheer scale of these costs, coupled with the trend of training compute doubling every five months and dataset sizes every eight months , reinforces the earlier point about the centralization of frontier AI development. Only organizations with access to massive capital resources can afford to play at this level.
3.3 Inference Economics: Falling Costs and API Pricing Models
In sharp contrast to the escalating costs of training frontier models, the cost of using these models for inference (i.e., generating predictions or responses) has been decreasing dramatically. This trend is a key enabler of broader AI adoption. As highlighted previously, the cost to query a model performing at the level of GPT-3.5 plummeted over 280-fold between late 2022 and late 2024. This reduction is driven by several factors: architectural optimizations leading to more efficient models (especially SLMs), improvements in hardware efficiency, algorithmic improvements in the inference process itself, and increasing competition among API providers.
The dominant pricing model for accessing LLMs via APIs is token-based, where users pay based on the amount of text processed (input tokens) and generated (output tokens). Typically, 1,000 tokens correspond to roughly 750 words. Pricing varies significantly based on the model's capability, provider, and whether it's input or output (output is often more expensive). Representative 2025 pricing per million tokens includes :
- GPT-4o (OpenAI): ~$5 input / ~$15 output
- Claude 3 Sonnet (Anthropic): ~$3 input (includes output)
- Gemini 2 Pro (Google): ~$3-$5 input (includes output)
- DeepSeek V3: ~$0.50-$1.50 input (includes output)
Costs for specialized tasks like creating embeddings (numerical representations of text used for search/RAG) are generally much lower, e.g., OpenAI's text-embedding-3-small
costs around $0.10 per million tokens. This pay-as-you-go model lowers the initial barrier for developers and businesses to integrate sophisticated AI capabilities, allowing them to scale costs with usage. However, for high-volume applications, these token costs can still accumulate rapidly and become a major recurring operational expense.
Reflecting a maturing market seeking different value propositions, alternative pricing models are also emerging :
- Output-Based Pricing: Charging based on the value delivered, e.g., per marketing paragraph generated (Copy.ai).
- Off-Peak Discounts: Offering lower prices for using models during non-peak hours (DeepSeek).
- Task-Specific Pricing: Charging per unit relevant to the task, e.g., per page processed for Document AI (Google Cloud, Azure AI Document Intelligence).
- Subscription Models: Offering tiered access or enhanced features for a flat monthly fee (ChatGPT Plus/Team, Amazon Alexa+).
These evolving pricing strategies aim to better align costs with perceived value, optimize resource utilization for providers, or bundle AI features into existing subscription services.
3.4 Beyond the Model: Infrastructure, Talent, and Operational Costs
The total cost of implementing and operating AI solutions extends far beyond model training or API fees. Several other significant cost categories must be considered for a complete economic picture.
- Infrastructure: Deploying AI, especially self-hosted open-source models or custom-trained systems, requires substantial infrastructure investment. Cloud platforms like AWS SageMaker, Azure ML, and Google AI Platform are widely used, with monthly costs ranging from $500 to over $10,000 depending heavily on compute usage (CPU/GPU instances), storage, and network traffic. Renting high-end GPUs (like NVIDIA A100s or H100s) needed for training or demanding inference tasks can cost $3 to $20+ per hour for each instance. While on-premise hardware offers potential long-term cost control, it involves significant upfront capital expenditure ($50k-$200k+) and ongoing maintenance. Specialized AI platforms (e.g., Dataiku, H2O AI) add another layer of potential software costs ($1k-$50k+/month). Vector databases, essential for RAG applications, typically add $20 to $500+ per month depending on scale.
- Talent: Acquiring and retaining skilled AI professionals remains a major expense due to high demand and specialized expertise. Approximate 2025 annual salary ranges in the US are substantial: AI Engineers ($100k-$200k), Data Scientists ($100k-$180k), Machine Learning Engineers ($120k-$220k), and AI Researchers (often PhD level) ($150k-$300k+). While outsourcing development to regions with lower labor costs (e.g., Eastern Europe, India) can offer savings (hourly rates of $30-$50+ vs. $50-$100+ in US/Europe), managing outsourced projects effectively requires careful planning and oversight.
- Integration: Connecting AI models or solutions into existing business processes and IT systems can be complex and costly, estimated at $10k to $100k or more. Legacy systems often lack modern APIs, and data silos may require significant restructuring, adding to the integration burden.
- Maintenance and Hidden Costs: AI systems are not "set and forget." They require ongoing monitoring, evaluation, and periodic retraining or fine-tuning to maintain performance as data drifts or business needs evolve. Several "hidden" costs can also inflate the total cost of ownership :
- Data Storage Sprawl: Abandoned experiments and model checkpoints can consume significant cloud storage indefinitely if not managed (e.g., 100 failed PyTorch experiments could cost ~$275/month in storage).
- Shadow IT: Unauthorized use of AI tools by different departments can lead to unaccounted cloud spending and security risks.
- Data Transfer Fees: Moving data between regions (e.g., processing European data in US-based models) can incur substantial egress charges.
- Compliance and Security: Ensuring AI systems meet regulatory requirements (like GDPR, HIPAA) and implementing robust security measures adds overhead.
This analysis reveals a bifurcated cost structure in the AI landscape. Creating frontier foundation models involves astronomical, concentrated costs accessible only to a few. Conversely, leveraging these existing models through APIs or open-source deployments is becoming increasingly affordable due to falling inference costs. This democratizes access to AI power. However, the total cost for organizations building AI applications remains significant when factoring in the necessary investments in infrastructure, specialized talent, data preparation, integration, and ongoing operational management. Sustainable AI implementation requires a holistic view of these lifecycle costs, careful planning, and robust cost management practices.
Section 4: Mapping the AI Market: Key Players and Offerings
The AI market in 2025 is a complex and dynamic ecosystem populated by established technology giants, critical infrastructure providers, and a burgeoning scene of innovative startups. Understanding the key players and their strategic positioning is crucial for navigating this landscape.
4.1 Industry Giants: Strategies of Major Tech Companies
Several large technology companies wield significant influence, leveraging their vast resources, existing customer bases, and research capabilities to shape the AI field. Their strategies often involve a mix of developing proprietary models, providing cloud platforms, integrating AI into existing products, and sometimes contributing to the open-source community.
- Microsoft: Deeply intertwined with OpenAI through massive investments , Microsoft aggressively integrates OpenAI's models (GPT-4o, DALL-E 3) across its product portfolio via Copilot in Microsoft 365, Windows, and other services. Its Azure AI platform offers comprehensive tools and access to various models (including OpenAI's) for developers. Simultaneously, Microsoft develops its own highly efficient Phi series of models, including reasoning and multimodal variants, contributing to the SLM trend and open-weight community. Microsoft's strategy hinges on leveraging its enterprise dominance and partnerships to embed AI deeply within user workflows.
- Google (Alphabet): A long-standing pioneer in AI research through DeepMind, Google's flagship offering is the Gemini family of models (Ultra, Pro, Flash, Nano), which are natively multimodal and integrated into core products like Google Search, Workspace, and Android. Google Cloud AI (Vertex AI) provides a robust platform for AI development and deployment. While Google has made seminal contributions to open research (e.g., the Transformer architecture) and frameworks (TensorFlow), its most advanced models like Gemini remain proprietary. Its strategy leverages its vast data resources, research prowess, and consumer reach.
- Meta: Has emerged as a primary champion of the open-source approach in the LLM space with its Llama series (Llama 2, Llama 3, 3.1, 3.2). By releasing powerful models openly, Meta aims to democratize access, accelerate community innovation, and challenge the dominance of closed models. It is also integrating AI features, like the Meta AI assistant, into its massive social media platforms (Facebook, Instagram, WhatsApp). Meta invests heavily in training infrastructure and develops the widely used PyTorch framework.
- Amazon: Primarily exerts influence through Amazon Web Services (AWS), the leading cloud infrastructure provider, which hosts a vast amount of AI development and deployment globally. AWS offers managed AI services like SageMaker (for building, training, deploying models) and Bedrock (providing access to a variety of foundation models from different providers). Amazon is also investing in custom AI silicon (Trainium for training, Inferentia for inference) to optimize performance and reduce costs for customers running AI workloads on AWS. Additionally, it integrates AI into its e-commerce operations and consumer devices (e.g., Alexa+ subscription).
- OpenAI: A leading force in AI research and deployment, known for pushing the boundaries with models like GPT-4, GPT-4o, o1 (reasoning), DALL-E (image generation), and Sora (video generation). Its strategy is primarily proprietary, offering access through its popular ChatGPT interface and extensive APIs. Its close partnership with Microsoft provides significant funding and infrastructure support. Despite facing intense competition, OpenAI remains highly influential and continues to seek massive funding for future development.
- Anthropic: Co-founded by former OpenAI members, Anthropic emphasizes AI safety and ethics alongside developing highly capable models like the Claude series (Haiku, Sonnet, Opus). Its approach is largely proprietary, focusing on controlled development and alignment techniques. It has secured substantial backing from Google and Amazon and is innovating in areas like agentic AI with "Computer Use" capabilities for Claude.
- IBM: Leverages its long history in enterprise computing, offering AI solutions through its IBM Watson platform. Focus areas include business process automation (Watson Orchestrate) and developer productivity (Watson Code Assistant).
- Salesforce: A leader in Customer Relationship Management (CRM), Salesforce integrates AI capabilities (Einstein GPT) deeply into its platform to enhance sales, service, and marketing functions, aiming to provide predictive insights and automate customer interactions.
- Apple: Traditionally more focused on user experience and privacy, Apple integrates AI features across its ecosystem (iOS, macOS), often prioritizing on-device processing using its custom silicon (Neural Engine). While historically less public about its large-scale model development, it is increasingly incorporating advanced AI capabilities.
- Chinese Giants (Baidu, Alibaba, Tencent): These companies are major forces, particularly within the large Chinese market and increasingly globally. Baidu is known for its Ernie family of LLMs and its leadership in autonomous driving with Apollo Go. Alibaba and Tencent are also investing heavily in AI, integrating it into their extensive cloud services, e-commerce platforms, and social/gaming applications.
Table 6: Major AI Players: Key Offerings & Focus Areas (2025)
Company | Core AI Models/Products | Cloud/Infra Offerings | Key Strategic Focus |
---|---|---|---|
Microsoft | Copilot (integrating OpenAI models), Phi Series (SLMs) | Azure AI Platform, Azure ML, Custom Silicon (Maia) | Enterprise Integration, Partnership (OpenAI), Hybrid AI |
Gemini Family (Ultra, Pro, Flash, Nano), Gemma (Open) | Google Cloud AI (Vertex AI), TPUs | Search/Data Advantage, Research Leadership, Proprietary | |
Meta | Llama Series (Open Source LLMs), Meta AI Assistant | PyTorch Framework, Own Training Infra | Open Source Leadership, Social Integration |
Amazon | Alexa+, (Access to various models via Bedrock) | AWS (SageMaker, Bedrock), Custom Silicon (Trainium/Inf) | Cloud Dominance, Infrastructure Optimization |
NVIDIA | (Provides platform for others) | GPUs (H100, Blackwell), CUDA, NIM Microservices | Hardware Leadership, AI Computing Platform |
OpenAI | GPT-4, GPT-4o, o1, DALL-E 3, Sora | (Relies on Azure) | Frontier Model Development, Proprietary API Access |
Anthropic | Claude Series (Haiku, Sonnet, Opus) | (Relies on AWS/GCP) | AI Safety & Alignment, Proprietary Models |
IBM | Watson Platform (Orchestrate, Code Assistant) | IBM Cloud | Enterprise Solutions, Business Automation |
Salesforce | Einstein GPT | (Runs on own/partner clouds) | CRM Integration, Business AI Applications |
4.2 Hardware and Cloud: The Enabling Infrastructure
The advancements in AI models are critically dependent on the underlying hardware and cloud infrastructure that enables their training and deployment at scale.
- NVIDIA: Remains the dominant force in AI hardware, providing the high-performance Graphics Processing Units (GPUs)-such as the H100 and the newer Blackwell architecture-that are the workhorses for training and running most large AI models. Beyond hardware, NVIDIA offers a comprehensive software ecosystem, including CUDA (its parallel computing platform), and increasingly, pre-built software containers and microservices like NVIDIA NIM (NVIDIA Inference Microservices) designed to simplify and accelerate the deployment of popular AI models. NVIDIA also provides specialized platforms targeting specific industries, like Clara for healthcare and Drive AGX for automotive. Its near-monopoly on high-end AI training chips makes it a company of significant geopolitical interest, particularly concerning supply chain dependencies and export controls.
- AMD: Is NVIDIA's primary competitor in the market for AI accelerators, offering its Instinct line of GPUs (including the MI300 series and the upcoming MI350) as alternatives for training and inference. AMD is steadily gaining market share and provides crucial competition in the high-performance computing space needed for AI.
- Cloud Providers (AWS, Azure, Google Cloud): These hyperscalers provide the essential backbone for much of the AI ecosystem. They offer scalable access to massive compute resources (including NVIDIA and AMD GPUs, as well as their own custom chips), storage, networking, and a suite of managed AI services (like Amazon SageMaker, Microsoft Azure AI Studio, and Google Vertex AI) that simplify the process of building, training, and deploying AI models. They are increasingly developing their own custom AI silicon-such as AWS's Trainium and Inferentia chips, Google's Tensor Processing Units (TPUs), and Microsoft's Maia accelerator-aimed at optimizing performance and reducing costs for AI workloads running on their specific platforms.
- Custom Silicon and Specialized Hardware Innovators: A growing number of companies are challenging the traditional GPU-centric approach by developing novel hardware architectures specifically tailored for AI computations. Cerebras Systems offers its unique Wafer Scale Engine (WSE) for large-scale AI training. SambaNova Systems and Groq focus on inference acceleration. Startups like Etched are designing chips (ASICs) specifically optimized for transformer inference, aiming for higher efficiency on specific workloads. Arm, whose energy-efficient CPU designs dominate the mobile market, is also crucial for data centers and edge AI deployments where power consumption is a key concern. Intel remains a major player in CPUs used alongside accelerators and is developing its own line of AI chips (Gaudi). Additionally, companies like Xscape Photonics are exploring the use of light (photons) instead of electrons for data processing, promising potential breakthroughs in speed and efficiency. This diversification in hardware offers the potential for more optimized solutions for specific AI tasks beyond general-purpose GPUs.
4.3 Innovators and Disruptors: The Vibrant Startup Scene
Beyond the established giants, the AI landscape in 2025 is characterized by a dynamic and well-funded startup ecosystem driving innovation across various segments. These startups often focus on specific niches, develop novel technologies, or challenge incumbents with different business models (Ref: Forbes AI 50 , CB Insights AI 100 , CRN AI 100 , other mentions ).
Key categories of AI startups include:
- Foundational Model Developers: Competing with or complementing the giants, startups like Cohere (often enterprise-focused) , Mistral AI (European leader, strong open-source presence) , Elon Musk's xAI (developing Grok) , DeepSeek (Chinese competitor) , Sakana AI (Japan, focusing on novel architectures) , Reka AI , and the stealthy Thinking Machine Labs (founded by former OpenAI CTO Mira Murati) are developing their own large language or multimodal models.
- AI Infrastructure, Platforms & Tools: This is a particularly active area. Hugging Face provides a crucial hub for the open-source community, hosting models, datasets, and tools. Databricks offers a unified platform for data engineering, analytics, and AI. LangChain provides essential tools for building LLM applications. Scale AI is a leader in data labeling and annotation necessary for training models. Others focus on vector databases (Pinecone, Weaviate) , synthetic data generation (Gretel) , specialized AI cloud providers (Lambda, Together AI, Crusoe) , or deployment platforms (Baseten, Fireworks AI).
- Enterprise & Vertical AI Applications: Many startups focus on applying AI to solve specific business problems or cater to particular industries. Glean offers AI-powered enterprise search. Harvey develops AI tools for the legal sector. Writer provides AI writing assistance tailored for enterprises. Adept and Sierra are building AI agents for customer service. Others target healthcare (Tempus AI, Abridge) , finance, manufacturing, or defense (Vannevar Labs). Palantir, while more established, provides data platforms heavily used by government and large enterprises.
- Consumer-Facing & Creative Tools: This segment has seen explosive growth. Perplexity AI offers an AI-native search engine experience. ElevenLabs leads in realistic voice cloning and generation. Midjourney, Pika, Runway, and Synthesia are popular tools for AI image and video generation/editing. Cursor (Anysphere) is a rapidly growing AI-first code editor. Speak provides AI-powered language tutoring. Suno generates music from prompts.
- Robotics & Physical AI: Startups like Figure AI are developing humanoid robots intended for labor tasks, attracting significant investment. Others like Skild AI focus on AI systems for controlling robotic systems.
This vibrant startup scene injects dynamism into the market, often pioneering new applications and pushing established players to innovate faster. The increasing number of AI startup IPOs (Initial Public Offerings), such as Astera Labs (connectivity), Rubrik (data management), Klaviyo (marketing AI), and Tempus AI (healthcare), with more highly anticipated candidates like Databricks and Anthropic on the horizon, signals a maturing market where AI-driven value is being recognized by public investors.
The AI market in 2025 is best understood not as a monolithic entity dominated by a few giants, but as a complex, interdependent ecosystem. Foundational model developers rely critically on hardware providers like NVIDIA and cloud platforms like AWS and Azure for the immense resources needed for training and, often, deployment. In turn, application developers-ranging from startups to established SaaS companies-leverage these foundational models (accessed via APIs or open-source releases) and utilize development tools and frameworks (like Hugging Face and LangChain) to build their specific products and services. The cloud providers benefit immensely from this activity, hosting both the demanding training workloads and the scalable deployment of countless AI applications, creating a powerful flywheel effect for their platforms. They often act as aggregators, offering access to models from multiple developers through services like AWS Bedrock or Azure AI Studio.
Within this ecosystem, strategic positioning is key. Competing directly on building the largest, most general-purpose foundational model is an incredibly expensive proposition feasible for only a handful of players. Consequently, many successful players, especially startups, find their niche by focusing on specific layers or applications: developing superior developer tools (e.g., Cursor, LangChain), providing critical infrastructure components (e.g., Scale AI for data, Pinecone for vector search), targeting specific industry verticals with tailored solutions (e.g., Harvey for legal, Tempus for healthcare), or creating novel consumer experiences built on AI (e.g., Perplexity, ElevenLabs). Even the giants differentiate: Microsoft leverages its enterprise software dominance, Google its search and data expertise, Meta its social reach and open-source strategy, and Amazon its cloud leadership. Partnerships also play a crucial role in navigating this interdependent landscape, exemplified by the Microsoft-OpenAI relationship, Google and Amazon's investments in Anthropic, and NVIDIA's collaborations with infrastructure partners like DDN. Success in 2025 often hinges on understanding these ecosystem dynamics and carving out a defensible strategic position within it.
Section 5: Trending AI Applications and Concepts in 2025
Beyond the underlying models and market players, 2025 is witnessing the rise and refinement of specific AI applications and conceptual shifts that are defining how AI is used and perceived. Key trends include the maturation of specific AI tools achieving product-market fit, the emergence of agentic AI systems capable of autonomous action, the increasing importance of multimodality, and the continued evolution of flagship model families.
5.1 Hottest AI Tools: Popularity and Product-Market Fit
The rapid innovation in AI has spawned a plethora of tools, but certain applications have gained significant traction with users, demonstrating strong product-market fit (PMF) by effectively solving specific problems or enhancing productivity. Examples of popular tools across categories in 2025 include:
- AI Chatbots & Assistants:
- ChatGPT (OpenAI): Remains a dominant force and often the benchmark, powered by models like GPT-4o and the reasoning-focused o1. Its flexibility and ease of use contribute to its wide adoption.
- Claude (Anthropic): Known for its focus on safety ("helpful, honest, and harmless") and strong performance in creative writing and coding tasks, with features like "Artifacts" for generating interactive outputs.
- Google Gemini: The successor to Bard, integrated across Google products, offering multimodal capabilities and strong performance.
- Microsoft Copilot: Integrated deeply into Microsoft's ecosystem (Windows, Office/365), leveraging OpenAI's latest models to provide contextual assistance within workflows. Used by over 1 million customers.
- Poe (Quora): An aggregator platform allowing users to access various models (Claude, DeepSeek, OpenAI) and create custom bots.
- AI-Powered Search Engines:
- Perplexity AI: Gaining significant attention for its conversational search interface that provides direct answers with cited sources, often seen as a complement or alternative to traditional search engines. Processes 169 million queries monthly.
- Google AI Overviews: Google's integration of AI-generated summaries directly into its main search results page.
- Content Generation (Image, Video, Voice, Music):
- Image: Midjourney remains popular for artistic image generation ; OpenAI's DALL-E (integrated into ChatGPT/Copilot) is also widely used.
- Video: Tools like Synthesia (AI avatars), Runway, Pika, and OpusClip are enabling easier video creation and editing.
- Voice: ElevenLabs is a leader in realistic text-to-speech and voice cloning, crossing 1 million users. Murf is another popular option.
- Music: Startups like Suno and Udio allow users to generate music from text prompts.
- Productivity, Design & Coding:
- Design: Canva's Magic Studio integrates various AI tools (text-to-image, magic design) into its popular graphic design platform, used by over 170 million active customers. Looka focuses on AI logo generation.
- Writing & Grammar: Grammarly continues to be a widely used AI writing assistant ; Rytr and Sudowrite cater to specific writing needs.
- Coding: GitHub Copilot is extremely popular among developers, with nearly half of code on the platform being AI-generated. Cursor (Anysphere) is an AI-first IDE experiencing rapid growth. Amazon Q Developer and Tabnine are other notable players.
- Knowledge Management: Notion AI Q&A allows users to query their own notes and documents.
- Translation: DeepL is renowned for its high-quality, nuanced translations across numerous languages, boasting massive organic traffic.
The success of these tools highlights several factors driving PMF in the AI era. Sheer technological capability is necessary but not sufficient. Winning products often excel at :
- Solving a Specific, High-Value Problem: Tools that clearly address user pain points (e.g., tedious research, writer's block, inefficient coding, language barriers) gain traction.
- Integration into Workflows: Tools that seamlessly fit into existing user workflows (like Copilot in Office, Canva's AI features, GitHub Copilot in IDEs) see faster adoption.
- Ease of Use: Intuitive interfaces (often conversational) lower the barrier to entry.
- Reliability and Trust: While hallucinations remain an issue , tools that provide reliable outputs or cite sources (like Perplexity) build user trust.
- Performance and Speed: Delivering results quickly enhances the user experience.
- Iterative Improvement: Successful AI startups often ship updates relentlessly and adapt quickly to user feedback and the changing technological landscape.
Finding PMF for AI products involves unique challenges. Founders must be wary of "fake PMF" driven by initial hype but undermined by high churn or unsustainable service models. Understanding the user's true "Job-To-Be-Done" and designing interfaces that go beyond simple chat are crucial. The trend is also moving from AI primarily automating analytical "left-brain" tasks towards assisting or collaborating on creative "right-brain" tasks, requiring different product design considerations. The concept of human-AI collaboration is evolving, shifting from distinct roles ("Centaurs") towards more integrated partnerships ("Cyborgs") where AI acts as an extension of human capabilities.
Table 7: Popular AI Tools & Primary Use Cases (2025)
Tool Name | Category | Primary Use Case / Value Proposition | Developer/Company (Example) |
---|---|---|---|
ChatGPT | Chatbot / AI Assistant | General purpose conversation, content generation, coding, Q&A | OpenAI |
Claude | Chatbot / AI Assistant | Creative writing, coding, safe/ethical responses, interactive outputs | Anthropic |
Google Gemini | Chatbot / AI Assistant | Multimodal interaction, integration with Google ecosystem, search assist | |
Microsoft Copilot | Chatbot / AI Assistant | Workflow integration (Office, Windows), contextual assistance | Microsoft (uses OpenAI) |
Perplexity AI | AI Search Engine | Conversational search with cited sources, research assistance | Perplexity AI |
Midjourney | Image Generation | High-quality, artistic image creation from text prompts | Midjourney Inc. |
ElevenLabs | Voice Generation / Cloning | Realistic text-to-speech, voice cloning, multilingual audio generation | ElevenLabs |
Synthesia / Runway | Video Generation / Editing | AI avatar creation, video editing, text-to-video | Synthesia / Runway ML |
GitHub Copilot | AI Coding Tool | Code completion, generation, debugging within IDEs | GitHub (Microsoft/OpenAI) |
Cursor | AI Coding Tool | AI-first code editor, natural language coding assistance | Anysphere |
DeepL | AI Translation | High-quality, nuanced machine translation across many languages | DeepL SE |
Canva Magic Studio | AI Graphic Design | Integrated AI tools (image gen, text gen) within popular design platform | Canva |
Notion AI Q&A | Knowledge Management | Querying and summarizing information within personal/team Notion workspaces | Notion |
Suno | Music Generation | Creating songs and audio tracks from text prompts | Suno AI |
5.2 The Rise of Agentic AI: Autonomous Systems at Work
One of the most significant conceptual and technological trends in AI for 2025 is the development and increasing application of Agentic AI. This represents a paradigm shift from earlier AI systems, which were largely reactive (responding to specific prompts or inputs), towards systems capable of proactive, autonomous action to achieve complex goals.
Agentic AI systems are defined by their ability to :
- Perceive: Gather information about their environment (digital or physical).
- Reason: Analyze the perceived information, understand context, and formulate plans or strategies to achieve a given objective.
- Act: Execute actions (e.g., interact with software, control hardware, communicate) based on their reasoning and plan.
- Learn: Adapt their behavior and improve performance over time based on feedback and experience, often utilizing reinforcement learning techniques.
Crucially, agentic AI operates with minimal human oversight or intervention once a goal is set. They can handle complex, multi-step tasks that require planning, decomposition, and dynamic adaptation to changing circumstances. This capability often arises from combining the flexible reasoning and language understanding abilities of LLMs with the precision and reliability of traditional software execution and potentially specialized AI models or tools. An agentic system might consist of multiple specialized AI agents, each responsible for a specific sub-task (e.g., data gathering, analysis, communication, execution), orchestrated by a central reasoning component (often a powerful LLM) that manages the overall goal and workflow.
The potential applications of agentic AI are vast and transformative, promising significant increases in productivity and automation across various domains :
- Business Process Automation: Automating complex workflows like financial audits, supply chain optimization (e.g., Amazon's system dynamically rerouting shipments based on real-time data) , or end-to-end insurance claims processing.
- Customer Service: Moving beyond simple chatbots to agents that can proactively resolve complex customer issues, manage appointments, and personalize interactions.
- Cybersecurity: Autonomous agents that can detect anomalies in network traffic, identify threats, and initiate countermeasures in real-time without human intervention (e.g., Darktrace).
- HR and Recruitment: AI agents screening candidates, analyzing interview responses (e.g., HireVue), or even conducting initial automated screening calls (e.g., Mercor).
- Software Development & IT Operations: Agents assisting with complex coding tasks, managing system maintenance, or automating deployment processes.
- Scientific Discovery: Agents designing experiments, analyzing data, and formulating hypotheses.
- Personal Assistants: More capable virtual assistants managing complex schedules, travel arrangements, or research tasks.
- Human-Computer Interaction: Agents that can understand high-level user intent and operate computer interfaces (GUIs) via mouse and keyboard control to complete tasks, exemplified by OpenAI's Computer-User Agent (CUA) / Operator.
The development of agentic AI is facilitated by frameworks like LangChain, Microsoft's AutoGen, and CrewAI, which provide tools for building, orchestrating, and managing these autonomous systems. While still an emerging field with challenges around reliability, safety, and control, agentic AI is considered a top technology trend for 2025 and represents the next frontier in AI-driven automation and intelligence.
5.3 Beyond Text: The Advance of Multimodal AI
Another crucial trend defining AI in 2025 is the rapid advancement and integration of Multimodal AI. Unlike earlier models that primarily focused on a single data type (usually text), multimodal systems are designed to process, understand, and generate information across multiple modalities, including text, images, audio, video, and potentially other sensor data (like physiological signals or environmental readings).
This ability to integrate diverse data types allows AI systems to develop a more holistic and contextually rich understanding of the world, mirroring human perception more closely. This leads to more natural interactions and enables entirely new classes of applications.
Major AI developers are actively incorporating multimodality into their flagship models:
- OpenAI: GPT-4V introduced powerful image understanding capabilities, allowing users to ask questions about images. The latest GPT-4o model enhances these capabilities and integrates audio processing. OpenAI's CUA (Computer-User Agent) uses vision (screenshots) as a primary input for interacting with graphical user interfaces. Their Sora model focuses on video generation.
- Google: The Gemini family of models was designed from the ground up to be natively multimodal, capable of reasoning seamlessly across text, images, audio, and code.
- Microsoft: Has released Phi-4-multimodal, a relatively small (5.6B parameters) but capable model that processes text, image, and audio inputs to generate text outputs.
- Meta: While Llama 3 is currently text-focused, Meta has explicitly stated plans for future versions to incorporate multimodal capabilities.
- Anthropic: Claude models are also incorporating multimodal features, including image understanding.
- Other Models: Specialized models like CogVLM are pushing boundaries in integrating text, image, and audio.
The capabilities enabled by multimodal AI are expanding rapidly:
- Enhanced Understanding: Models can analyze images, charts, and documents containing both text and visuals, extracting deeper insights.
- Richer Interaction: Users can interact with AI using voice, images, and text simultaneously.
- Cross-Modal Generation: Generating text descriptions from images, generating images or code from text and visual inputs (e.g., creating a webpage based on a sketch) , or generating video from text.
- Sensor Fusion: Integrating data from various sensors (visual, auditory, lidar, physiological) for applications in autonomous systems (self-driving cars) , robotics, and healthcare (e.g., analyzing medical images alongside patient records and biosignals).
- Emotion Recognition: Analyzing facial expressions, voice tone, and physiological signals to understand human emotional states for applications in mental health, user experience research, or human-robot interaction.
Despite the rapid progress, significant challenges remain in multimodal AI development. These include sourcing high-quality, diverse, and well-aligned multimodal datasets; developing effective and efficient techniques for fusing information from different modalities; addressing modality incompleteness (missing data types); managing privacy risks associated with sensitive multimodal data; and ensuring the deployability, safety, and interpretability of these complex systems, particularly in high-stakes applications. Research focus is increasingly shifting from purely model-centric development towards addressing these data-centric and deployment-centric challenges to unlock the full potential of multimodal AI for real-world impact.
5.4 Model Spotlights: Llama 3, Phi-4, Mistral/Mixtral Deep Dives
Examining specific leading model families provides concrete examples of the broader trends discussed. Llama 3, Phi-4, and the Mistral/Mixtral models each exemplify key directions in AI development in 2025.
- Llama 3 (Meta): As Meta's flagship open-source offering, Llama 3 represents the power of large-scale training and open release. Available in 8B and 70B parameter versions (with a 400B+ model trained and potentially forthcoming), it was pre-trained on an enormous dataset of over 15 trillion tokens sourced from publicly available data, significantly larger than its predecessor, Llama 2. Meta emphasized rigorous data filtering and quality control, even using Llama 2 itself to help generate training data for quality classifiers. Key architectural improvements include a larger vocabulary (128,000 tokens for efficiency), a doubled context length (8,192 tokens compared to Llama 2), and the use of Grouped Query Attention (GQA) for optimized inference. Llama 3 demonstrates strong performance across standard benchmarks like MMLU, HumanEval, and MATH, showing significant improvements in reasoning capabilities, code generation, and the ability to follow complex instructions compared to Llama 2. Its capabilities support a wide range of applications, including advanced conversational AI, content generation, language translation, and data analysis. While currently text-focused, Meta plans future multilingual and multimodal versions. Safety measures include tools like Llama Guard 2 and CyberSec Eval 2 for responsible deployment.
- Phi-4 Series (Microsoft): This family showcases the trend towards highly capable Small Language Models (SLMs). Starting with Phi-1 and Phi-2, Microsoft demonstrated that models trained on carefully curated, high-quality "textbook-like" data could achieve remarkable performance despite smaller sizes. The Phi-3-mini (3.8B parameters) achieved performance comparable to much larger models on benchmarks like MMLU. The Phi-4 generation builds on this, with a 14B parameter base model and specialized variants. Phi-4-reasoning (14B) is fine-tuned specifically for complex reasoning tasks using curated prompts and demonstrations generated by larger models (like OpenAI's o3-mini), showing significant gains in math, coding, and planning benchmarks, outperforming models 5x its size. Phi-4-reasoning-plus uses further reinforcement learning for even higher accuracy, albeit with longer generation times. Phi-4-multimodal (5.6B) integrates text, image, and audio processing into a single compact model. A key focus for the Phi series is efficiency and deployability, including optimizations for running on edge devices and PCs (e.g., Phi Silica for Copilot+ PCs). The models also support function calling, enabling interaction with external tools and APIs. Robust safety post-training using SFT, DPO, and RLHF techniques is employed across the family.
- Mistral / Mixtral Models (Mistral AI): This European startup is known for pushing the boundaries of performance and efficiency, often through open-weight releases. Mistral 7B gained popularity as a highly capable compact model. The Mixtral models (8x7B and the larger 8x22B) employ a Sparse Mixture-of-Experts (SMoE) architecture. In an SMoE model, the input is routed to only a subset ("experts") of the model's parameters for processing, rather than activating the entire network like a traditional dense model. For Mixtral 8x22B, this means activating only 39 billion of its total 141 billion parameters during inference, leading to significantly faster inference speeds and lower computational cost compared to a dense model of similar capability. Both Mistral 7B and Mixtral models are often released under permissive licenses like Apache 2.0. Mixtral 8x22B boasts strong multilingual capabilities (English, French, Italian, German, Spanish), robust math and coding skills, and a large 64k token context window. Mistral AI also offers a proprietary flagship model, Mistral Large (estimated 123B parameters ), which competes at the top tier of performance benchmarks, offering strong multilingual reasoning, a 32k token context window, and native function calling capabilities. Fine-tuning experiments show that Mixtral models offer substantial performance gains over Mistral 7B for complex tasks, although the simpler model may suffice for less demanding applications. Mistral's models are accessible via their own platform, cloud marketplaces (like Azure), and increasingly through deployment frameworks like NVIDIA NIM.
These three model families encapsulate key strategic directions in 2025: Meta's Llama demonstrates the power of scaled open-source releases; Microsoft's Phi highlights the potential of data curation and efficient SLMs; and Mistral AI's models showcase architectural innovation (SMoE) and European competitiveness in both open and proprietary spaces.
5.5 AI in Action: Top Enterprise Use Cases
While cutting-edge research explores agentic AI and advanced reasoning, the practical application of AI in enterprises during 2025 is largely focused on leveraging current generative AI capabilities, primarily LLMs, to drive efficiency and enhance existing processes. An analysis of 530 real-world generative AI projects implemented by enterprises between 2022 and 2024 provides valuable insights into current adoption patterns.
The analysis reveals that generative AI is being applied broadly across various business functions, with the top five departments being Customer Support, Marketing, IT, Operations, and R&D. The technology sector itself is the leading adopter, accounting for 56% of the identified projects, often developing AI-powered products (like SAP's Joule or Salesforce Einstein) that are then sold to other industries. Manufacturing (8%) and Professional Services (7%) follow as the next largest adopting industries. Geographically, North America leads implementation with 56% of projects.
The top 10 specific enterprise applications identified, based on their frequency among the 530 projects, are :
- Issue Resolution for Customer Support (35%): Using AI agents or chatbots to handle inbound customer tickets, complaints, and problems. Example: Klarna's AI customer service agent handling inquiries 24/7 across multiple markets.
- Information Retrieval for Customer Support (14%): Enabling customers or support staff to quickly find information in manuals, knowledge bases, or documentation. Example: Kuka Xpert using Empolis Buddy to answer product-specific questions.
- Content Creation for Marketing (11%): Generating marketing copy, blog posts, social media updates, email campaigns, and internal communications.
- Code Generation/Assistance for R&D/IT (9%): Utilizing AI coding assistants integrated into IDEs (like GitHub Copilot, Amazon Q Developer, JetBrains AI Assistant) to speed up software development, suggest code, find bugs, and answer programming questions.
- Document Summarization (Internal) (7%): Automatically condensing long reports, research papers, meeting transcripts, or emails for faster consumption.
- Knowledge Management Search (Internal) (6%): Improving the ability to search and retrieve information from internal company knowledge bases and repositories.
- Sales Outreach Personalization (5%): Using AI to help tailor sales emails, messages, and pitches to individual prospects based on their profiles or needs.
- Market Research Analysis (4%): Applying AI to analyze large volumes of market data, competitor information, or customer feedback to identify trends and insights.
- HR Candidate Screening/Summarization (3%): Using AI to parse resumes, screen candidates based on requirements, and summarize applicant profiles.
- Predictive Maintenance Analysis (Manufacturing) (2%): Analyzing sensor data from industrial equipment to predict potential failures and schedule maintenance proactively.
Other significant enterprise use cases gaining traction include: enhancing personalized marketing strategies beyond content generation , optimizing supply chain logistics and inventory management , improving financial operations through predictive analytics and advanced fraud detection , enhancing quality control processes in manufacturing , and accelerating product development cycles using generative AI for ideation or prototyping. AI agents are increasingly seen as a key enabling technology across many of these applications.
The current prevalence of these specific applications suggests a pragmatic approach by enterprises in 2025. The most widely adopted use cases tend to be those where current generative AI, particularly LLMs, offers clear and demonstrable value in automating or augmenting text-heavy, often repetitive tasks. Areas like customer support, marketing content, and developer assistance represent "low-hanging fruit" where the technology's strengths align well with business needs, and implementation, while still challenging, is often more straightforward than overhauling core operational systems or deploying high-risk autonomous decision-making. The more transformative applications envisioned with advanced agentic AI, complex reasoning, or physical world interaction are certainly on the horizon and being actively developed, but they likely represent the next wave of enterprise adoption, building upon the foundation and experience gained from these initial, more grounded deployments.
Table 8: Top Enterprise AI Applications & Adoption Insights (2025)
Rank | Use Case | % of Projects* | Key Departments | Example Tools/Companies Mentioned |
---|---|---|---|---|
1 | Issue Resolution (Customer Support) | 35% | Customer Support | Klarna AI Agent, AI Chatbots |
2 | Information Retrieval (Cust. Support) | 14% | Customer Support | Kuka Xpert (Empolis Buddy), Knowledge Base AI |
3 | Content Creation (Marketing) | 11% | Marketing | GenAI Writing Tools |
4 | Code Generation/Assistance | 9% | R&D, IT | GitHub Copilot, Amazon Q Dev, JetBrains AI Assistant |
5 | Document Summarization (Internal) | 7% | Various | LLM Summarization Tools |
6 | Knowledge Management Search (Internal) | 6% | Various | Enterprise Search AI (e.g., Glean), Notion AI Q&A |
7 | Sales Outreach Personalization | 5% | Sales | AI Sales Tools |
8 | Market Research Analysis | 4% | Marketing, Strategy | AI Data Analysis Tools |
9 | HR Candidate Screening/Summarization | 3% | Human Resources | AI Recruitment Tools (e.g., Textio, CVViZ, Mercor) |
10 | Predictive Maintenance Analysis | 2% | Manufacturing, Ops | AI Industrial Monitoring |
* Based on analysis of 530 enterprise generative AI projects implemented 2022-2024, reported Feb 2025.
Conclusion
The state of AI in 2025 is one of dynamic acceleration and deepening integration. Performance continues to leap forward, not just incrementally but often dramatically on complex benchmarks, while the competitive frontier becomes increasingly crowded. Simultaneously, remarkable gains in model efficiency, particularly the rise of powerful small language models, coupled with plummeting inference costs, are making sophisticated AI capabilities more accessible than ever before. This economic shift is fueling a surge in enterprise adoption, moving AI from the periphery to a central role in business strategy, although unlocking its full potential often requires challenging workflow transformations rather than superficial implementation.
The global landscape remains led by the US in terms of investment and frontier model development, but China is rapidly closing the performance gap, and innovation is becoming more distributed globally. This is significantly aided by a thriving open-source ecosystem, where models like Llama 3, Phi-4, and Mistral/Mixtral offer compelling alternatives to proprietary systems, fostering collaboration and providing advantages in cost, customization, and privacy. This creates a productive tension with the highly centralized and expensive process of training the absolute largest and most capable foundation models, leading to a bifurcated economic structure.
Trending concepts like agentic AI, promising autonomous goal achievement, and multimodal AI, enabling richer understanding across data types, point towards the next wave of transformation. However, current popular tools and widespread enterprise applications often reflect a pragmatic focus on leveraging today's generative AI for tangible efficiency gains in text-based tasks like customer support, content creation, and coding assistance.
Navigating this era requires acknowledging the concurrent rise in AI-related risks and incidents. The gap between the pace of technological advancement and the development of robust governance frameworks-both within organizations and through regulation-remains a critical challenge. As AI becomes more powerful and pervasive, the imperative for responsible development, ethical considerations, effective risk management, and transparent practices intensifies. For businesses, policymakers, and society at large, strategic adaptation, continuous learning, and a commitment to balancing innovation with thoughtful governance are essential to harness the profound potential of AI while mitigating its inherent complexities in the years ahead.
About Baytech
At Baytech Consulting, we specialize in guiding businesses through this process, helping you build scalable, efficient, and high-performing software that evolves with your needs. Our MVP first approach helps our clients minimize upfront costs and maximize ROI. Ready to take the next step in your software development journey? Contact us today to learn how we can help you achieve your goals with a phased development approach.
About the Author
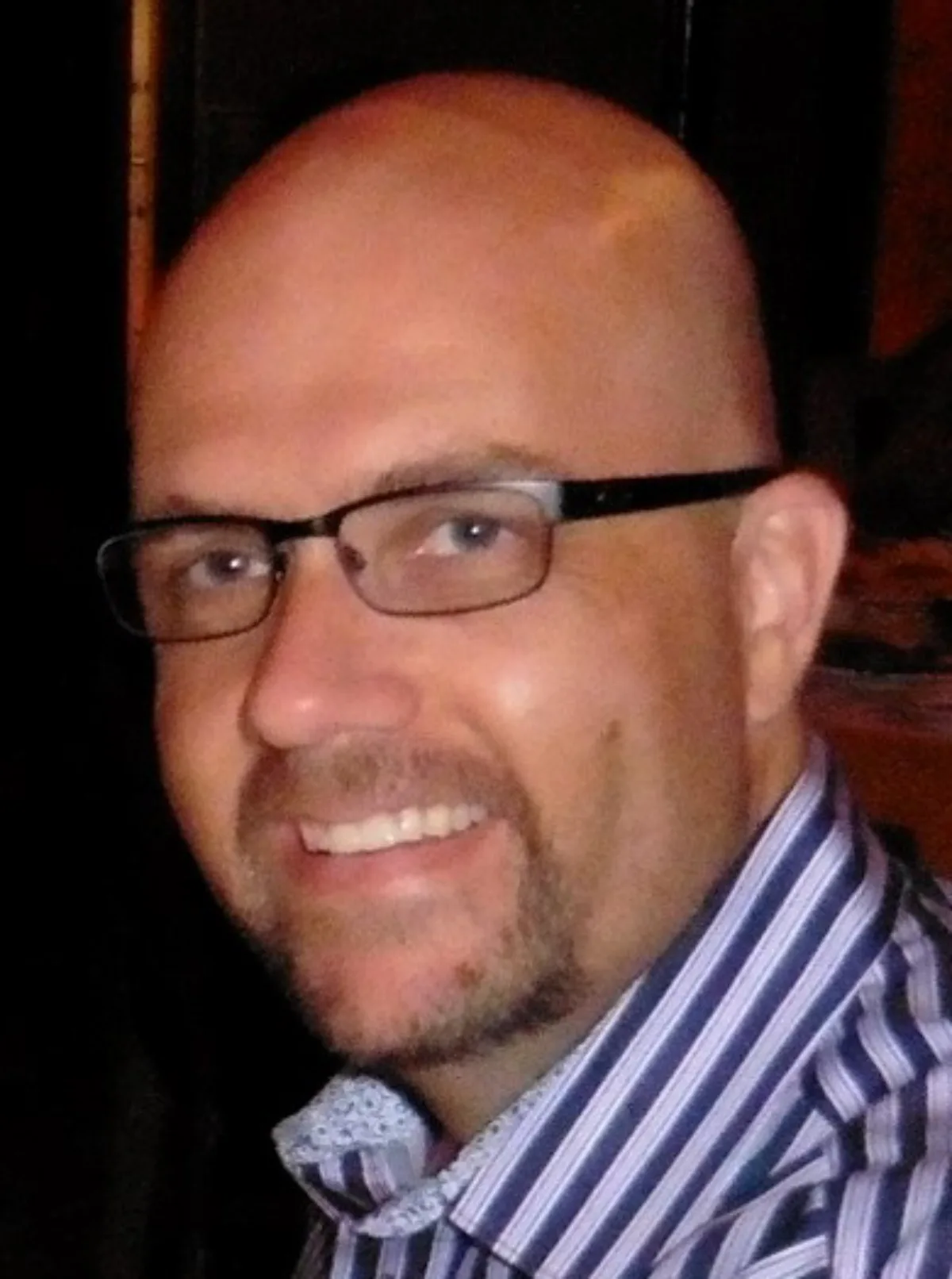
Bryan Reynolds is an accomplished technology executive with more than 25 years of experience leading innovation in the software industry. As the CEO and founder of Baytech Consulting, he has built a reputation for delivering custom software solutions that help businesses streamline operations, enhance customer experiences, and drive growth.
Bryan’s expertise spans custom software development, cloud infrastructure, artificial intelligence, and strategic business consulting, making him a trusted advisor and thought leader across a wide range of industries.